A SAR Automatic Target Recognition Method Based on Capsule Network
An automatic target recognition and capsule technology, which is applied in the field of SAR automatic target recognition based on deep learning, can solve problems affecting image feature extraction, and achieve the effect of improving performance.
- Summary
- Abstract
- Description
- Claims
- Application Information
AI Technical Summary
Problems solved by technology
Method used
Image
Examples
Embodiment Construction
[0035] The implementation method of the content of the invention herein will be described in detail in order to better reflect the technical gist of the invention. The present invention is a SAR target recognition method based on a convolutional capsule network, and each step is specifically implemented in the following manner.
[0036] Step 1: Cut the collected SAR image into 64*64 slices to reduce the influence of redundant background on feature extraction;
[0037] Step 2: Perform preliminary feature learning through a convolutional layer with a kernel size of 3*3, and obtain 32 feature maps of 60*60;
[0038] Step 3: Use multiple convolution kernels with different expansion rates to extract features of different scales. The expansion convolution used in the present invention is used to extract features of different scales. Specifically, the size of the convolution kernel is 5×5, and the expansion ratios are 2, 3, and 5 respectively;
[0039] Step 4: Input multi-scale fea...
PUM
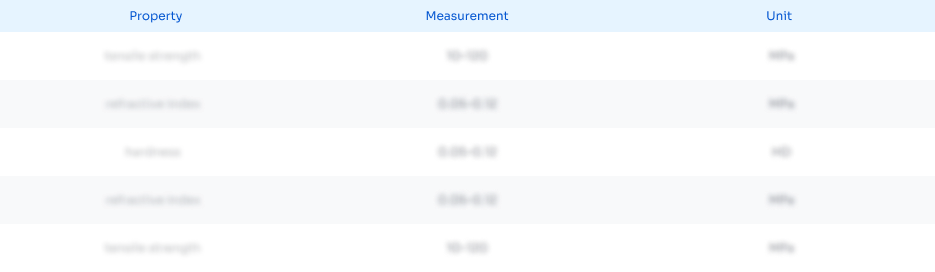
Abstract
Description
Claims
Application Information
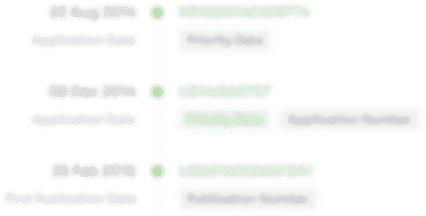
- R&D
- Intellectual Property
- Life Sciences
- Materials
- Tech Scout
- Unparalleled Data Quality
- Higher Quality Content
- 60% Fewer Hallucinations
Browse by: Latest US Patents, China's latest patents, Technical Efficacy Thesaurus, Application Domain, Technology Topic, Popular Technical Reports.
© 2025 PatSnap. All rights reserved.Legal|Privacy policy|Modern Slavery Act Transparency Statement|Sitemap|About US| Contact US: help@patsnap.com