Load prediction method under extreme scene based on modal decomposition and transfer learning
A technology of transfer learning and forecasting method, which is applied in the field of load forecasting in extreme scenarios based on modal decomposition and transfer learning, which can solve the problems of no reference value of historical data, sudden change of power load, and inability to calculate more accurate forecast values by time series methods, etc. , to achieve the effect of solving the endpoint effect
- Summary
- Abstract
- Description
- Claims
- Application Information
AI Technical Summary
Problems solved by technology
Method used
Image
Examples
Embodiment Construction
[0031] In this example, if figure 1 As shown, a load forecasting method for extreme scenarios based on modal decomposition and transfer learning is carried out as follows:
[0032] Step 1. Statize the historical data of power load in various extreme scenarios, and classify according to the trend of the load curve to obtain the historical data of power load with classification labels; then collect the historical data in the scenarios to be predicted;
[0033] Specifically, extreme scenarios include natural disasters such as earthquakes, mountain torrents, mudslides, typhoons, frosts, or uncontrollable factors such as equipment failures that cause abnormal load changes; Sampling the load power value at a uniform time in normal state;
[0034] Step 2. Use the improved aggregation empirical mode decomposition method to decompose the historical data of the scene to be predicted and the historical data of the same type in the historical data of electric load with classification lab...
PUM
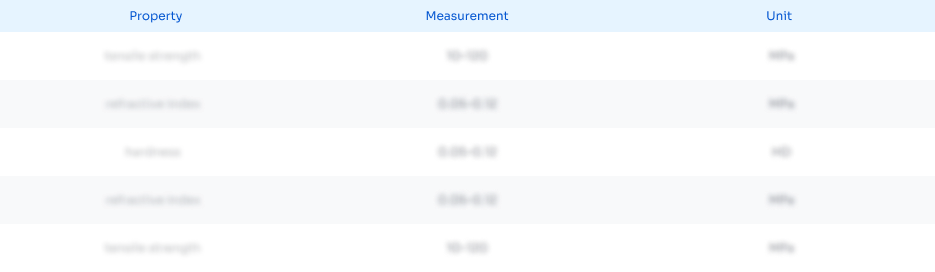
Abstract
Description
Claims
Application Information
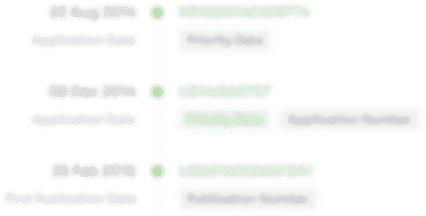
- R&D
- Intellectual Property
- Life Sciences
- Materials
- Tech Scout
- Unparalleled Data Quality
- Higher Quality Content
- 60% Fewer Hallucinations
Browse by: Latest US Patents, China's latest patents, Technical Efficacy Thesaurus, Application Domain, Technology Topic, Popular Technical Reports.
© 2025 PatSnap. All rights reserved.Legal|Privacy policy|Modern Slavery Act Transparency Statement|Sitemap|About US| Contact US: help@patsnap.com