Load characteristic rapid matching method based on AdaBoost model
A technology of load characteristics and matching methods, applied in character and pattern recognition, data processing applications, instruments, etc., can solve problems such as low efficiency of mathematical optimization solutions, difficult to meet, and simple processing scenarios, and achieve good economic benefits and practical value , to achieve a simple, high-accuracy effect
- Summary
- Abstract
- Description
- Claims
- Application Information
AI Technical Summary
Problems solved by technology
Method used
Image
Examples
Embodiment 1
[0077] Such as figure 1 Shown, the present invention comprises the following steps:
[0078] 1) Acquire data, the data includes training data, label data, weak recognizer and number of iterations; the training data is the graphic feature data in the load V-I curve trajectory; the training data and the label data are input into the training data set to form a training data set, wherein the training The dataset is:
[0079] T={(x 1 ,y 1 ),(x 2 ,y 2 ),...,(x n ,y n )} (1)
[0080] In the formula: T is the training data set; x i is the load characteristic; y i It is the label corresponding to the load feature. The label refers to the working state of the equipment. When it is -1, it means it is in a stopped state. When it is 1, it means it is working;
[0081] 2) Initialize the weight distribution of the weak discriminator;
[0082]
[0083] In the formula: D 1 To initialize the weight distribution set of training samples, w i,n is the n-th training weight of the i...
Embodiment 2
[0101] Embodiment two: the same place as the embodiment will not be repeated, the difference is:
[0102] Such as image 3 As shown, the data acquisition of the graphic feature data in the load V-I curve track includes the following steps:
[0103] 101) Acquisition of total electricity load data;
[0104] Use the wave recorder to collect the voltage and current data of a single electrical appliance, and obtain the drawn VI trajectory curve;
[0105] 102) data preprocessing;
[0106] Data preprocessing includes standardization of current and voltage; standardization of current and voltage is achieved by dividing the current and voltage signals by their root mean squares respectively; the standardized current and voltage data are plotted with the voltage as the abscissa axis and the current as the ordinate axis to draw the current Voltage V-I trajectory curve;
[0107] The current and voltage normalized calculation formula is:
[0108]
[0109] v norm(n) =v n / V rms (...
PUM
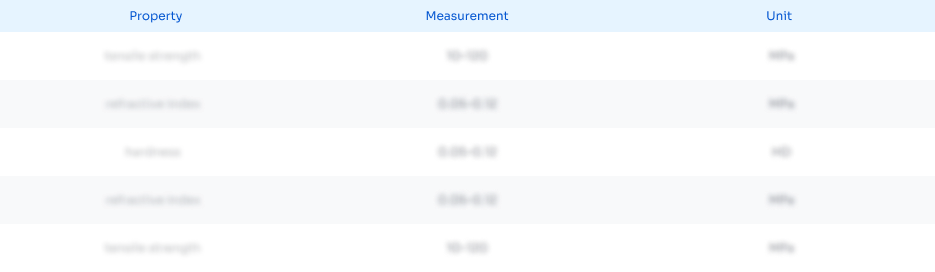
Abstract
Description
Claims
Application Information
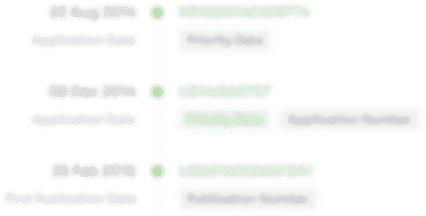
- R&D Engineer
- R&D Manager
- IP Professional
- Industry Leading Data Capabilities
- Powerful AI technology
- Patent DNA Extraction
Browse by: Latest US Patents, China's latest patents, Technical Efficacy Thesaurus, Application Domain, Technology Topic, Popular Technical Reports.
© 2024 PatSnap. All rights reserved.Legal|Privacy policy|Modern Slavery Act Transparency Statement|Sitemap|About US| Contact US: help@patsnap.com