Robustness traffic flow prediction method based on multitask graph convolutional network
A convolutional network and prediction method technology, applied in the field of intelligent transportation and deep learning, can solve the problems of high-precision collected data instability, lack of traffic information, abnormalities, etc., and achieve the effect of overcoming tolerance and improving robustness
- Summary
- Abstract
- Description
- Claims
- Application Information
AI Technical Summary
Problems solved by technology
Method used
Image
Examples
Embodiment Construction
[0034] The technical solution of the present invention will be specifically described below in conjunction with the accompanying drawings.
[0035] A traffic flow robustness prediction method based on deep learning of the present invention is specifically implemented according to the following steps,
[0036] Step S1: Analyze the temporal-spatial correlation of the traffic network based on geographic information;
[0037] In this example, the Pearson correlation coefficient is used as an index to measure the correlation between the various modes of the data. It is found that the data has weekly correlation, daily correlation, time correlation and spatial correlation.
[0038] Step S2: Combine the graph convolution GCN (Graph Convolutional Networks) type with the multi-task learning MTL (Multi-Task Learning) to form a multi-task graph convolution model MTGCN (Multi-Task Graph Convolutional Networks), and train multiple tasks at the same time, Make the model more robust; as fo...
PUM
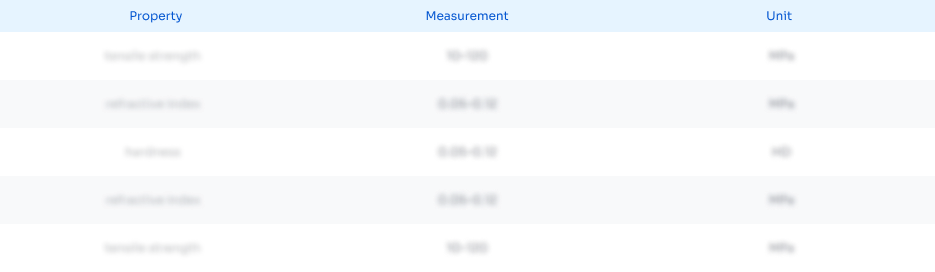
Abstract
Description
Claims
Application Information
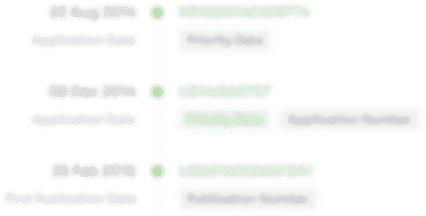
- R&D Engineer
- R&D Manager
- IP Professional
- Industry Leading Data Capabilities
- Powerful AI technology
- Patent DNA Extraction
Browse by: Latest US Patents, China's latest patents, Technical Efficacy Thesaurus, Application Domain, Technology Topic, Popular Technical Reports.
© 2024 PatSnap. All rights reserved.Legal|Privacy policy|Modern Slavery Act Transparency Statement|Sitemap|About US| Contact US: help@patsnap.com