End-edge collaborative federation learning optimization method based on edge calculation
An edge computing and optimization method technology, applied in neural learning methods, computing, machine learning, etc., can solve the problems of high computing overhead, too many updates and training times, and high global aggregation communication delay, so as to reduce communication delay and speed up The effect of training speed and reducing the number of global aggregations
- Summary
- Abstract
- Description
- Claims
- Application Information
AI Technical Summary
Problems solved by technology
Method used
Image
Examples
Embodiment 1
[0033] like Figure 1 to Figure 2 As shown, an end-edge collaborative federated learning optimization method based on edge computing includes the following steps: S1: Construct a federated learning optimization system architecture, which includes a cloud data center, an edge server, and a mobile terminal that are sequentially connected by communication; S2 : The mobile terminal and the edge server cooperate to update the global network model of the cloud data center locally; S3: After the local update is completed, the edge server gets the updated model parameters and sends them to the cloud data center for global aggregation to obtain a new global network model ; S4: The cloud data center conducts a model accuracy test on the new global network model.
[0034] In the above solution, in the architecture of the federated learning optimization system, the traditional two-layer architecture of device-cloud is changed to a three-layer architecture of device-edge-cloud, which mainl...
PUM
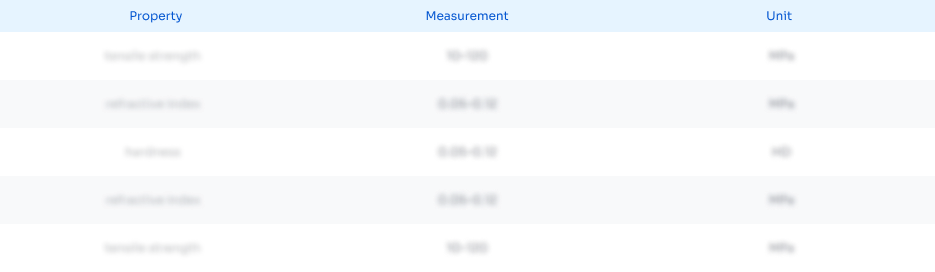
Abstract
Description
Claims
Application Information
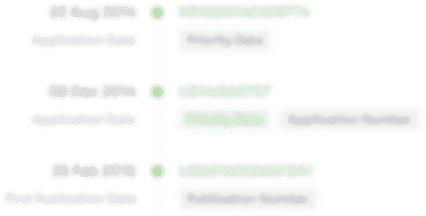
- Generate Ideas
- Intellectual Property
- Life Sciences
- Materials
- Tech Scout
- Unparalleled Data Quality
- Higher Quality Content
- 60% Fewer Hallucinations
Browse by: Latest US Patents, China's latest patents, Technical Efficacy Thesaurus, Application Domain, Technology Topic, Popular Technical Reports.
© 2025 PatSnap. All rights reserved.Legal|Privacy policy|Modern Slavery Act Transparency Statement|Sitemap|About US| Contact US: help@patsnap.com