Hyperspectral classification method based on deep feedforward network
A hyperspectral classification and feed-forward network technology, applied in the field of hyperspectral image classification, can solve the problems of high cost, time-consuming labeling of hyperspectral data samples, and difficult implementation
- Summary
- Abstract
- Description
- Claims
- Application Information
AI Technical Summary
Problems solved by technology
Method used
Image
Examples
Embodiment
[0072] The specific steps of the hyperspectral classification method based on deep feedforward network implemented by the present invention are as follows:
[0073] A. Sample data source: The hyperspectral data comes from the hyperspectral data of the IndianPine experimental area in Indian State, USA provided by Purdue University. The data has 220 bands and the size is 145×145×220. A total of 16 types of target objects are included, and the total number of target pixels is 10249. Its false color composite image as figure 2 As shown, the feature category labels are as follows image 3 shown.
[0074] Table 1 shows the number of samples for each type of target features:
[0075] Table 1
[0076]
[0077] B. First, set the training sample sampling rate to 5%. Under the condition that the total number of target pixels is 10249, calculate the total number of training samples to be allocated according to the formula (1) Then, use the training sample allocation algorithm (...
PUM
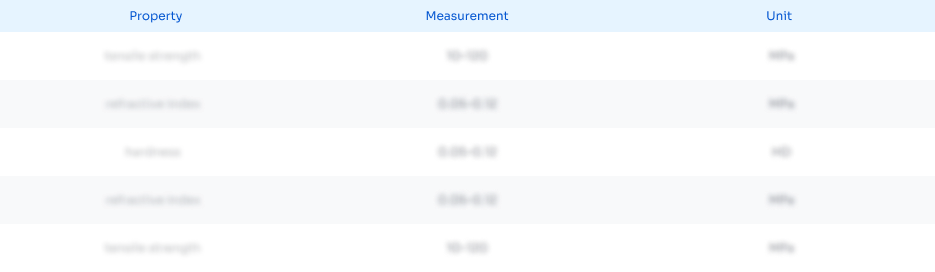
Abstract
Description
Claims
Application Information
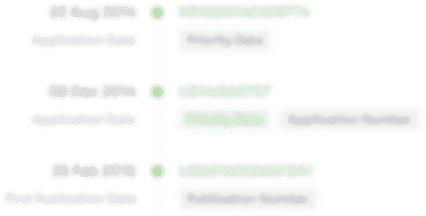
- R&D
- Intellectual Property
- Life Sciences
- Materials
- Tech Scout
- Unparalleled Data Quality
- Higher Quality Content
- 60% Fewer Hallucinations
Browse by: Latest US Patents, China's latest patents, Technical Efficacy Thesaurus, Application Domain, Technology Topic, Popular Technical Reports.
© 2025 PatSnap. All rights reserved.Legal|Privacy policy|Modern Slavery Act Transparency Statement|Sitemap|About US| Contact US: help@patsnap.com