Semantic segmentation method based on feature pyramid attention and mixed attention cascading
A feature pyramid and semantic segmentation technology, applied in the field of pattern recognition, can solve the problems of low segmentation accuracy, difficult to meet the requirements of segmentation accuracy, poor processing of segmentation target edge details, etc., to achieve the effect of optimizing processing and ensuring the speed of inference
- Summary
- Abstract
- Description
- Claims
- Application Information
AI Technical Summary
Problems solved by technology
Method used
Image
Examples
Embodiment Construction
[0055] A semantic segmentation method based on feature pyramid attention and mixed attention cascade, the specific steps are:
[0056] Step 1. Construct a semantic segmentation training set, specifically:
[0057] Preprocess the images in the Cityscapes urban road dataset, and perform normalization and standardization according to the RGB mean (0.485, 0.456, 0.406) and variance (0.229, 0.224, 0.225) of the dataset. 2975 finely labeled images are used as the training set, and 500 A finely annotated image is used as the validation set.
[0058] Step 2. Construct a deep convolutional neural network. The overall structure is as follows figure 2 Shown:
[0059] The deep convolutional neural network includes an encoder part, a feature pyramid attention module, a mixed attention module, a feature fusion part, and a decoding branch.
[0060] In a further embodiment, the encoder part adopts the structure in the existing MobileNetV2, such as image 3 As shown in a, the present inve...
PUM
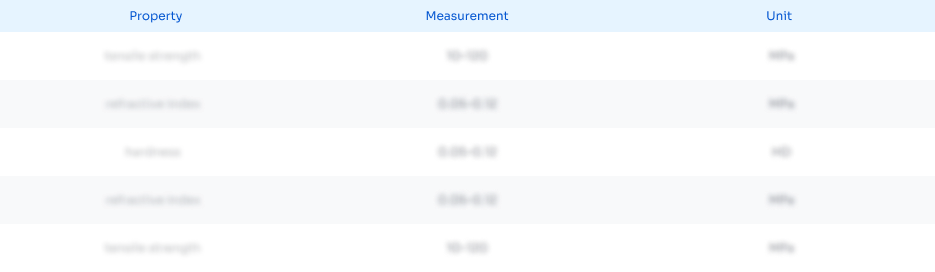
Abstract
Description
Claims
Application Information
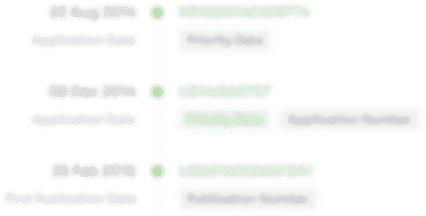
- R&D
- Intellectual Property
- Life Sciences
- Materials
- Tech Scout
- Unparalleled Data Quality
- Higher Quality Content
- 60% Fewer Hallucinations
Browse by: Latest US Patents, China's latest patents, Technical Efficacy Thesaurus, Application Domain, Technology Topic, Popular Technical Reports.
© 2025 PatSnap. All rights reserved.Legal|Privacy policy|Modern Slavery Act Transparency Statement|Sitemap|About US| Contact US: help@patsnap.com