No-reference image quality monitoring method based on channel recombination and feature fusion
A reference image and quality monitoring technology, applied in image enhancement, image analysis, image data processing, etc., can solve the problems of inconsistent results with human eyes, insufficient data samples, etc., achieve high consistency and ensure the effect of spatial integrity
- Summary
- Abstract
- Description
- Claims
- Application Information
AI Technical Summary
Problems solved by technology
Method used
Image
Examples
Embodiment 1
[0033] The embodiment of the present invention provides a no-reference quality monitoring algorithm based on channel reorganization and feature fusion, such as figure 1 As shown, the method includes the following steps:
[0034] 101: Preprocessing the image;
[0035] Preprocess the image, convert it into a grayscale image and divide it into non-overlapping small image blocks as input samples, and use a graph-based saliency analysis algorithm (Graph-based Visual Saliency, GBVS) to calculate each original image The significance score of the block.
[0036] 102: Perform feature extraction on the processed image;
[0037] Feature extraction is performed on input samples using a convolutional neural network. The input of the network is the 32×32 image block obtained in step 101 . Each input image block undergoes feature extraction to obtain a feature map with a size of 4×4×128.
[0038] 103: Reorganize and segment the extracted features in the channel dimension;
[0039] For ...
Embodiment 2
[0046] The scheme in embodiment 1 is further introduced below in conjunction with specific calculation formulas and examples, see the following description for details:
[0047] 201: image preprocessing;
[0048] Compute the saliency matrix for each image. Each pixel is assigned a value ranging from 0 to 255, with higher saliency values indicating a salient image pixel. The grayscale image and the corresponding saliency matrix are segmented into image patches of size 32×32. Based on the saliency matrix, the saliency score of each image patch is calculated by the following formula:
[0049]
[0050] In formula (1), S(m,n) is the saliency value of a pixel at position (m,n) in the i-th image block. M and N represent the size of the image block. The saliency score reflects the degree to which the image block attracts people's attention. The higher the saliency score of an image patch, the greater its impact on human judgment.
[0051] 202: Perform feature extraction on ...
Embodiment 3
[0068] Below in conjunction with concrete experiment, the scheme in embodiment 1 and 2 is carried out feasibility verification, see the following description for details:
PUM
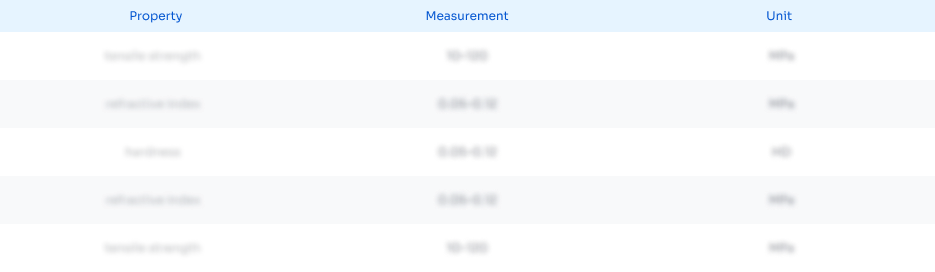
Abstract
Description
Claims
Application Information
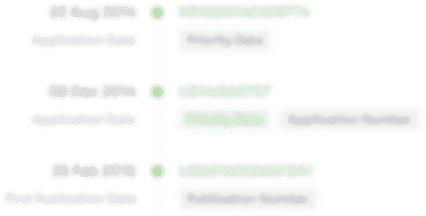
- R&D Engineer
- R&D Manager
- IP Professional
- Industry Leading Data Capabilities
- Powerful AI technology
- Patent DNA Extraction
Browse by: Latest US Patents, China's latest patents, Technical Efficacy Thesaurus, Application Domain, Technology Topic, Popular Technical Reports.
© 2024 PatSnap. All rights reserved.Legal|Privacy policy|Modern Slavery Act Transparency Statement|Sitemap|About US| Contact US: help@patsnap.com