Method for acquiring ultra-light classification network model
A classification network and acquisition method technology, applied in the field of classification network model acquisition, can solve the problems of time-consuming hardware configuration training, low accuracy of wide network, and expensive dependence on deep network.
- Summary
- Abstract
- Description
- Claims
- Application Information
AI Technical Summary
Problems solved by technology
Method used
Examples
Embodiment Construction
[0026] The present invention will be further described in detail below in combination with specific embodiments.
[0027] A method for obtaining an ultra-lightweight classification network model. Based on specific classification tasks, the neural network architecture search is performed to ensure the acquisition of a high-precision lightweight network b1; the method of fusion layer cutting and network pruning further cuts out the b1 network For worthless filters, get b3; then integrate TensorRT's graph optimization method to further accelerate the inference speed, and at the same time use the quantization method to further reduce the weight of the model, so as to obtain the final ultra-lightweight, ultra-fast and high-precision classification network model. Specifically include the following steps.
[0028] A. Based on the NAS neural network architecture search method, perform network search for specific classification tasks and obtain backbone->b1.
[0029] A1. Define the se...
PUM
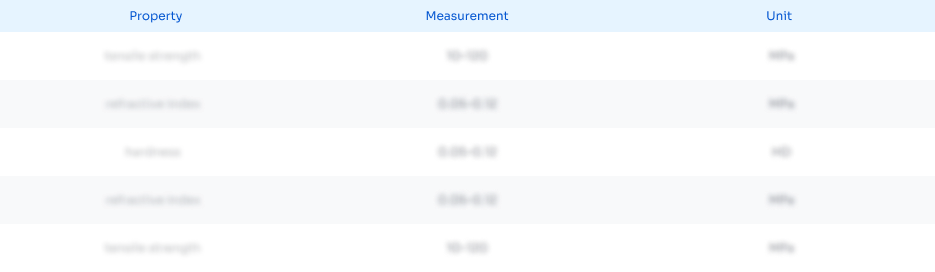
Abstract
Description
Claims
Application Information
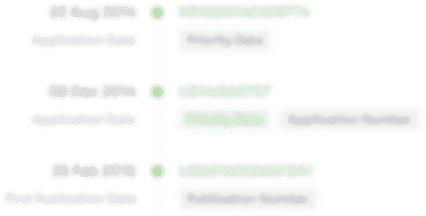
- R&D
- Intellectual Property
- Life Sciences
- Materials
- Tech Scout
- Unparalleled Data Quality
- Higher Quality Content
- 60% Fewer Hallucinations
Browse by: Latest US Patents, China's latest patents, Technical Efficacy Thesaurus, Application Domain, Technology Topic, Popular Technical Reports.
© 2025 PatSnap. All rights reserved.Legal|Privacy policy|Modern Slavery Act Transparency Statement|Sitemap|About US| Contact US: help@patsnap.com