Remote sensing image fusion method of multi-scale attention deep convolutional network based on 3D convolution
A deep convolution and attention technology, applied in the information field, can solve the problems of poor fusion quality and fusion effect, incomplete remote sensing image fusion, etc.
- Summary
- Abstract
- Description
- Claims
- Application Information
AI Technical Summary
Problems solved by technology
Method used
Image
Examples
Embodiment
[0107] In this example, two kinds of satellite remote sensing images are used to verify the effectiveness of the proposed fusion algorithm; the spatial resolutions of panchromatic images and multispectral images captured by IKONOS satellites are 1 meter and 4 meters respectively; panchromatic images provided by QuickBird satellites The spatial resolutions of the image and the multispectral image are 0.7 meters and 2.8 meters respectively; among them, the multispectral images acquired by the two satellites include four bands of red, green, blue and near-infrared; the size of the panchromatic image used in the experiment is 256 ×256, and the multispectral image size is 64×64.
[0108] In order to better evaluate the practicability of the remote sensing image fusion method (MASC-Net) of the multi-scale attention depth convolution network based on 3D convolution in this embodiment, this embodiment provides two types of experiments, which are respectively simulated image The experi...
PUM
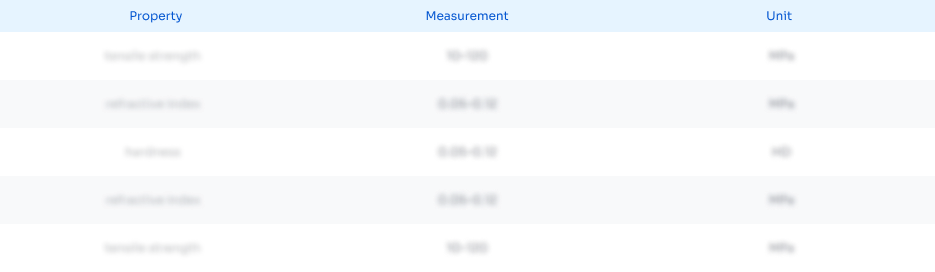
Abstract
Description
Claims
Application Information
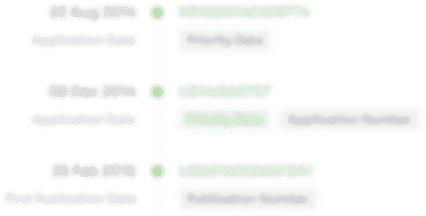
- Generate Ideas
- Intellectual Property
- Life Sciences
- Materials
- Tech Scout
- Unparalleled Data Quality
- Higher Quality Content
- 60% Fewer Hallucinations
Browse by: Latest US Patents, China's latest patents, Technical Efficacy Thesaurus, Application Domain, Technology Topic, Popular Technical Reports.
© 2025 PatSnap. All rights reserved.Legal|Privacy policy|Modern Slavery Act Transparency Statement|Sitemap|About US| Contact US: help@patsnap.com