A risk prediction method for aortic dissection surgery based on boosted tree model
A technology for aortic dissection and surgical risk, applied in the fields of medical data mining, character and pattern recognition, instruments, etc., can solve problems such as incompleteness, lack, and incomplete data, and achieve high accuracy.
- Summary
- Abstract
- Description
- Claims
- Application Information
AI Technical Summary
Problems solved by technology
Method used
Image
Examples
Embodiment 1
[0046] Decision tree algorithm is a traditional machine learning algorithm.
[0047] The core idea of the algorithm is to establish a set of trees to judge the judgment conditions based on the input features of the training set; each leaf node stores a category, and a set of multiple leafless nodes can contain multiple categories. The decision-making process is to classify samples into a category based on different feature values; the key point of building a decision tree is how to determine the direction of the next branch when it is at a certain node; the category of the split attribute is to divide all subsets as much as possible belong to the same category. Therefore, the core problem of the decision tree is how to choose the eigenvalue of the root node. Depending on the continuous or divisive nature of the output variable, decision trees can be classified as classification trees or regression trees.
[0048] The steps to build a decision tree are as follows:
[0049]...
PUM
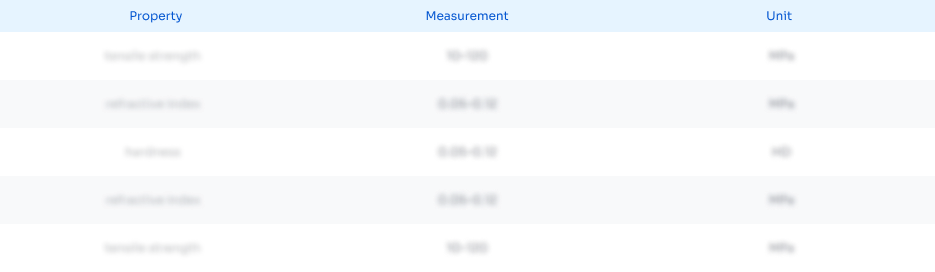
Abstract
Description
Claims
Application Information
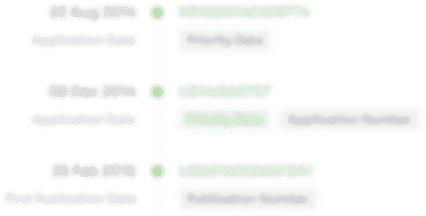
- R&D Engineer
- R&D Manager
- IP Professional
- Industry Leading Data Capabilities
- Powerful AI technology
- Patent DNA Extraction
Browse by: Latest US Patents, China's latest patents, Technical Efficacy Thesaurus, Application Domain, Technology Topic, Popular Technical Reports.
© 2024 PatSnap. All rights reserved.Legal|Privacy policy|Modern Slavery Act Transparency Statement|Sitemap|About US| Contact US: help@patsnap.com