Reservoir scheduling multi-objective optimization method based on graph convolutional neural network and NSGA-II algorithm
A convolutional neural network and multi-objective optimization technology, applied in neural learning methods, biological neural network models, neural architectures, etc., can solve problems that are not conducive to Pareto frontier convergence speed, increase computational complexity, and complex selection operations, etc., to achieve Strong global search ability, poor improvement of local search, wide application effect
- Summary
- Abstract
- Description
- Claims
- Application Information
AI Technical Summary
Problems solved by technology
Method used
Image
Examples
Embodiment Construction
[0041] The invention is a multi-objective optimization method for reservoir dispatching based on a graph convolutional neural network and NSGA-II algorithm, which optimizes the discharge volume of the reservoir in each time period, and obtains a non-inferior scheme set for the multi-objective optimization problem of reservoir dispatching. Collect data related to reservoir flood control scheduling and establish a multi-objective optimization model for reservoir scheduling; use the NSGA-Ⅱ algorithm to obtain the first-generation population of multi-objective optimization problems for reservoir scheduling, group the population individuals through coding operations, and mark categories, each category is used as A node of the GCN graph structure, the parent-child relationship obtained by the crossover and mutation operations is mapped to the edges between the GCN graph structure nodes, and the GCN graph structure and the preliminary Pareto front are obtained; the abscissa of the obta...
PUM
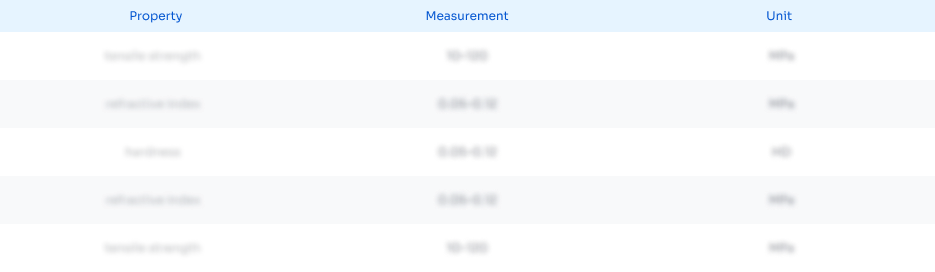
Abstract
Description
Claims
Application Information
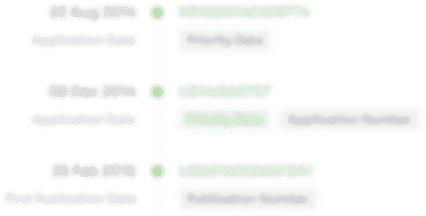
- Generate Ideas
- Intellectual Property
- Life Sciences
- Materials
- Tech Scout
- Unparalleled Data Quality
- Higher Quality Content
- 60% Fewer Hallucinations
Browse by: Latest US Patents, China's latest patents, Technical Efficacy Thesaurus, Application Domain, Technology Topic, Popular Technical Reports.
© 2025 PatSnap. All rights reserved.Legal|Privacy policy|Modern Slavery Act Transparency Statement|Sitemap|About US| Contact US: help@patsnap.com