Electrical load prediction method and system based on K-means clustering and BI-LSTM neural network
A technology of neural network and forecasting method, which is applied in the field of electrical load forecasting based on k-means clustering and BI-LSTM neural network, which can solve the problems of low electrical load forecasting accuracy, no hyperparameter optimization model, etc., and achieve forecasting reliability High, low impact, high reliability effect
- Summary
- Abstract
- Description
- Claims
- Application Information
AI Technical Summary
Problems solved by technology
Method used
Image
Examples
Embodiment Construction
[0035] The technical solutions of the present invention will be clearly and completely described below in conjunction with the embodiments of the present invention. Apparently, the described embodiments are only some of the embodiments of the present invention, not all of them. Based on the embodiments of the present invention, all other embodiments obtained by persons of ordinary skill in the art without creative efforts fall within the protection scope of the present invention.
[0036] The purpose of the present invention is to consider the factors affecting the change of electric load of the power grid through clustering, by analyzing the correlation between these multi-dimensional and complex data and historical electric load data, and then using the optimal parameter combination to obtain the BI-LSTM neural network prediction model, and then based on The optimal prediction model predicts the user's electricity load in a certain period of time in the future, see figure 1 ...
PUM
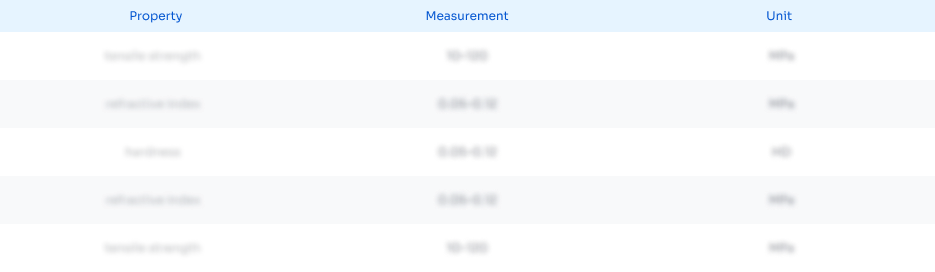
Abstract
Description
Claims
Application Information
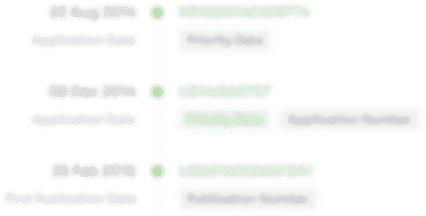
- R&D Engineer
- R&D Manager
- IP Professional
- Industry Leading Data Capabilities
- Powerful AI technology
- Patent DNA Extraction
Browse by: Latest US Patents, China's latest patents, Technical Efficacy Thesaurus, Application Domain, Technology Topic, Popular Technical Reports.
© 2024 PatSnap. All rights reserved.Legal|Privacy policy|Modern Slavery Act Transparency Statement|Sitemap|About US| Contact US: help@patsnap.com