Efficient asynchronous federated learning method for reducing communication times
A technology of communication frequency and learning method, which is applied in the field of efficient asynchronous federated learning, can solve the problems of large communication volume and model parameters, and achieve the effects of reducing communication times, fast convergence, and accelerated training
- Summary
- Abstract
- Description
- Claims
- Application Information
AI Technical Summary
Problems solved by technology
Method used
Image
Examples
Embodiment Construction
[0042] In order to clearly and completely describe the technical solutions in the embodiments of the present invention, the present invention will be further described in detail below in conjunction with the drawings in the embodiments.
[0043] The high-efficiency asynchronous federated learning flow chart of the embodiment of the present invention, such as figure 1 shown, including the following steps:
[0044] Step 1 deploys the model, and the parameter server pulls users to participate in training.
[0045] Step 1-1 When the training starts, deploy the model to be trained on the parameter server, and set the model version number to 0. This experiment uses a simple convolutional neural network for 10 classifications on the MNIST dataset, and evenly distributes the 60,000 training samples of the MNIST dataset to 100 users participating in federated learning.
[0046] Step 1-2 In this experiment, the number of users participating in federated learning is set to 100, and 10 ...
PUM
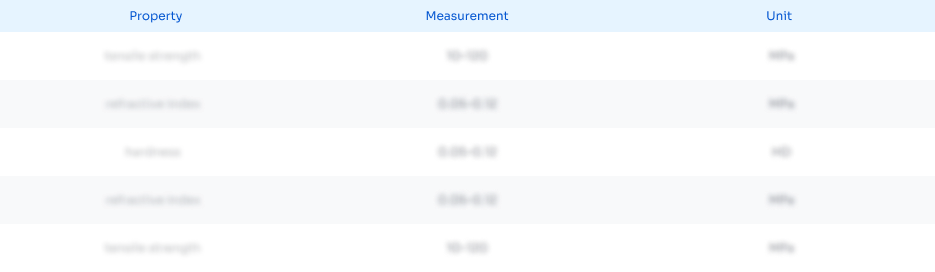
Abstract
Description
Claims
Application Information
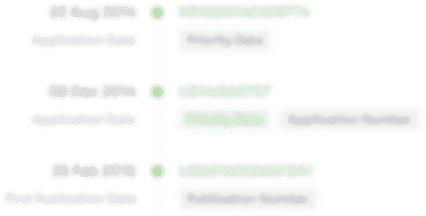
- R&D
- Intellectual Property
- Life Sciences
- Materials
- Tech Scout
- Unparalleled Data Quality
- Higher Quality Content
- 60% Fewer Hallucinations
Browse by: Latest US Patents, China's latest patents, Technical Efficacy Thesaurus, Application Domain, Technology Topic, Popular Technical Reports.
© 2025 PatSnap. All rights reserved.Legal|Privacy policy|Modern Slavery Act Transparency Statement|Sitemap|About US| Contact US: help@patsnap.com