A Machining Deformation Control Method Based on Meta Reinforcement Learning
A technology of reinforcement learning and processing deformation, applied in the direction of comprehensive factory control, comprehensive factory control, program control, etc., can solve problems such as increasing the number of sample data, and achieve the effect of reducing demand, realizing online optimization, and improving generalization ability.
- Summary
- Abstract
- Description
- Claims
- Application Information
AI Technical Summary
Problems solved by technology
Method used
Image
Examples
Embodiment Construction
[0031] The present invention will be further described below with reference to the accompanying drawings and examples, but the present invention is not limited to this embodiment.
[0032] like Figure 1-3 shown.
[0033] A meta-reinforcement learning-based finishing allowance optimization method includes the following steps:
[0034] 1. Taking the processing of aerospace structural parts as an example, during the processing of the parts, the deformation state of the workpiece is characterized by monitoring the deformation force of the workpiece.
[0035] 2. The deformation control process optimization method based on meta-reinforcement learning of the present invention, such as figure 1 shown. Firstly, the establishment of the reinforcement learning base model is analyzed, and the initial residual stress reference value of each layer of the blank is constructed according to the measurement results of the initial residual stress of the blank material of the part. where n ...
PUM
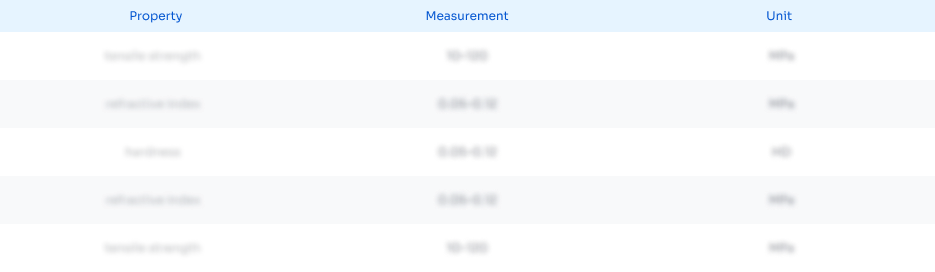
Abstract
Description
Claims
Application Information
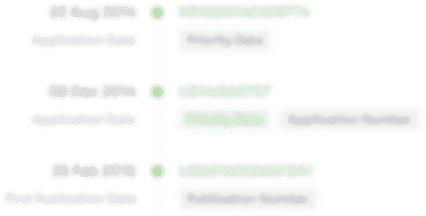
- R&D
- Intellectual Property
- Life Sciences
- Materials
- Tech Scout
- Unparalleled Data Quality
- Higher Quality Content
- 60% Fewer Hallucinations
Browse by: Latest US Patents, China's latest patents, Technical Efficacy Thesaurus, Application Domain, Technology Topic, Popular Technical Reports.
© 2025 PatSnap. All rights reserved.Legal|Privacy policy|Modern Slavery Act Transparency Statement|Sitemap|About US| Contact US: help@patsnap.com