A social robot detection method based on variational autoencoder and k-nearest neighbor combination
A detection method and robot technology, applied in the field of anomaly detection, can solve problems such as large differences, high-cost labeling and unbalanced positive and negative samples
- Summary
- Abstract
- Description
- Claims
- Application Information
AI Technical Summary
Problems solved by technology
Method used
Image
Examples
Embodiment Construction
[0010] like figure 2 As shown in the figure, the present invention provides a social robot detection method based on a combination of variational autocoding and anomaly detection. The steps of the invention method include: step 1, data acquisition and preprocessing, using a program to process the original text data obtained from the network to obtain the original feature matrix; step 2, feature generation by deep generative model variational auto-encoding; step 3, after feature fusion of original features and generated features, an anomaly detection method is used to detect social robots.
[0011] Step 1. Data acquisition and preprocessing, using the program to process the original text data obtained in the network to obtain the original feature matrix
[0012] There is very little public social robot data. The present invention selects the public CLEF2019 data set. This data set has labels, including 2880 training sets, 1240 verification sets, 100 tweets per account, and all...
PUM
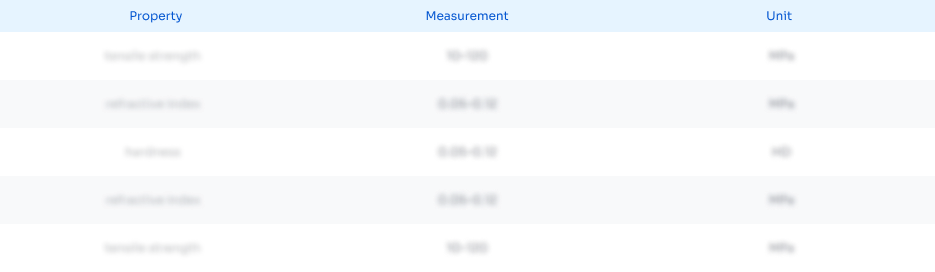
Abstract
Description
Claims
Application Information
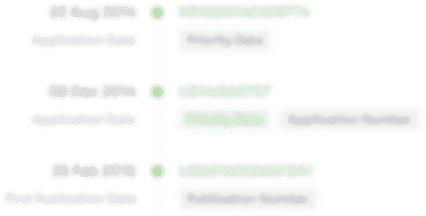
- R&D
- Intellectual Property
- Life Sciences
- Materials
- Tech Scout
- Unparalleled Data Quality
- Higher Quality Content
- 60% Fewer Hallucinations
Browse by: Latest US Patents, China's latest patents, Technical Efficacy Thesaurus, Application Domain, Technology Topic, Popular Technical Reports.
© 2025 PatSnap. All rights reserved.Legal|Privacy policy|Modern Slavery Act Transparency Statement|Sitemap|About US| Contact US: help@patsnap.com