Pedestrian re-identification method based on mixed cluster center label learning and storage medium
A pedestrian re-identification and cluster center technology, applied in the field of pedestrian re-identification, can solve the problems of poor generalization, inconsistent update ratio, outliers cannot be assigned pseudo-labels, etc., to achieve enhanced compactness, improved accuracy, and enhanced model feature learning The effect of the process
- Summary
- Abstract
- Description
- Claims
- Application Information
AI Technical Summary
Problems solved by technology
Method used
Image
Examples
Embodiment 1
[0047] A person re-identification method based on hybrid cluster center label learning, such as figure 2 , image 3 shown, including the following steps:
[0048] Step S100: collecting a pedestrian re-identification training data set, and dividing the training data set into labeled data samples and unlabeled data samples;
[0049] Step S200: first use the labeled data to initialize the parameters of the deep neural network model, and calculate the initial cluster center label and store it in the memory, and then use the deep neural network model to extract the feature information of the unlabeled data;
[0050] Step S300: Use multiple cluster center label generation modules to calculate the distance between the feature information of the unlabeled data and the cluster center and filter out the pseudo-label data with a preset ratio, and the remaining data are fuzzy label data and pseudo-label data Generating the cluster center label with the fuzzy label data as a guide label, ...
Embodiment 2
[0059] This embodiment is optimized on the basis of Embodiment 1. In the step S400, unlabeled data is added in a small number of iterations, and a small number of unlabeled data is added to the deep neural network model for training. , each iteration adds less unlabeled data than the previous iteration.
[0060] Further, the added unlabeled data increases at a rate of 10% for the first 10 epochs, and increases at a rate of 1% in subsequent iterations.
[0061] The above-mentioned small number of iterations is introduced into the model training process because the growth rate of the number of unlabeled data in the training data will affect the recognition accuracy of the overall network model. Added to the network model for training, the unlabeled data added in each iteration will be less than the data added last time, and the overall capacity of the training samples will be increased, thereby enhancing the performance of the model. The first 10 epochs of the added unlabeled da...
Embodiment 3
[0064] This embodiment is optimized on the basis of embodiment 1 or 2, as figure 1 As shown, the front end of the deep neural network model is provided with a block layer, which is used to divide the pedestrian image into three equal parts; the deep neural network model includes a convolution layer, a batch normalization layer, an activation Function layer, residual module, neuron deactivation layer, fully connected layer.
[0065] Further, the deep neural network model adopts a 64-layer residual deep neural network model.
[0066] The front end of the deep convolutional neural network builds a block layer to divide the pedestrian image into three equal parts, which is beneficial to the subsequent extraction of the feature information of each component. The backbone part adopts a residual network model with a 64-layer structure. The structure is to stack the convolutional layer, batch normalization layer, activation function layer, residual module, neuron deactivation layer,...
PUM
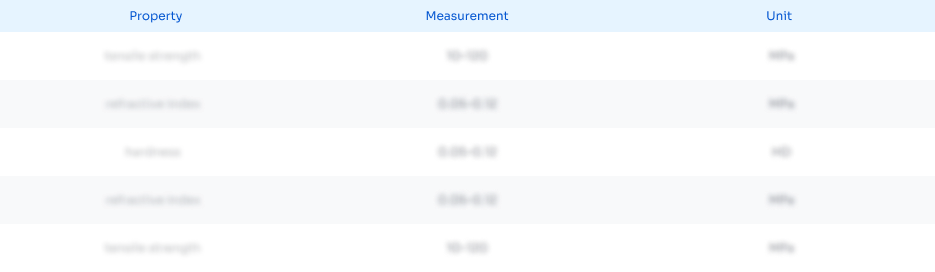
Abstract
Description
Claims
Application Information
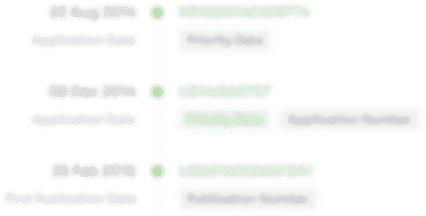
- R&D Engineer
- R&D Manager
- IP Professional
- Industry Leading Data Capabilities
- Powerful AI technology
- Patent DNA Extraction
Browse by: Latest US Patents, China's latest patents, Technical Efficacy Thesaurus, Application Domain, Technology Topic, Popular Technical Reports.
© 2024 PatSnap. All rights reserved.Legal|Privacy policy|Modern Slavery Act Transparency Statement|Sitemap|About US| Contact US: help@patsnap.com