Combined sparse signal dimension reduction gradient tracking reconstruction algorithm based on compressed sensing theory
A combined sparse and compressed sensing technology, applied in electrical components, code conversion, etc., can solve problems such as reducing computational complexity, increasing computational complexity, and reducing reconstruction accuracy, reducing computational complexity and improving reconstruction success. The effect of improving the efficiency and reconstruction power of
- Summary
- Abstract
- Description
- Claims
- Application Information
AI Technical Summary
Problems solved by technology
Method used
Image
Examples
Embodiment Construction
[0069] The specific implementation manners of the present invention will be described in detail below in conjunction with the accompanying drawings and examples.
[0070] Such as figure 1 Shown is the basic structure of compressed sampling based on compressed sensing theory. For signals that can be sparsely represented, this structure compresses the data while sampling the signal, which not only retains the key data required for signal recovery, but also alleviates the sampling system. Pressure, which combines data acquisition and compression into one, and the sampled data is restored through a signal reconstruction algorithm. The invention belongs to the technical field of analog information conversion (AIC) based on compressive sensing theory, and its basic principle is to multiply the random sequence of the hopping frequency greater than the Nyquist frequency by using the analog signal with sparse characteristics, and then through the integrator to The modulated signal is ...
PUM
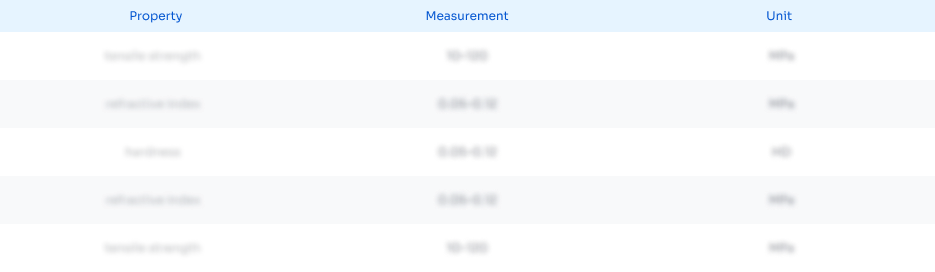
Abstract
Description
Claims
Application Information
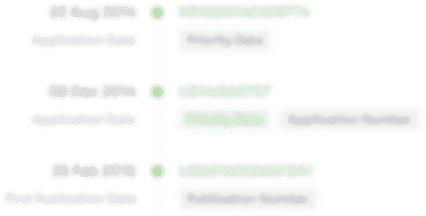
- Generate Ideas
- Intellectual Property
- Life Sciences
- Materials
- Tech Scout
- Unparalleled Data Quality
- Higher Quality Content
- 60% Fewer Hallucinations
Browse by: Latest US Patents, China's latest patents, Technical Efficacy Thesaurus, Application Domain, Technology Topic, Popular Technical Reports.
© 2025 PatSnap. All rights reserved.Legal|Privacy policy|Modern Slavery Act Transparency Statement|Sitemap|About US| Contact US: help@patsnap.com