Deep reinforcement learning model poisoning detection method and device based on time sequence neural pathway
A technology of reinforcement learning and detection methods, applied in neural learning methods, biological neural network models, neural architectures, etc., can solve problems such as being easily poisoned and difficult to detect, and achieve the effects of effective poisoning attacks, effective detection, and good applicability
- Summary
- Abstract
- Description
- Claims
- Application Information
AI Technical Summary
Problems solved by technology
Method used
Image
Examples
Embodiment Construction
[0024] In order to make the objectives, technical solutions and advantages of the present invention clearer, the present invention will be further described in detail below with reference to the accompanying drawings and embodiments. It should be understood that the specific embodiments described herein are only used to explain the present invention, and do not limit the protection scope of the present invention.
[0025] The technical idea of the present invention is as follows: in view of the problem that the deep reinforcement learning model is difficult to detect after being maliciously poisoned, the embodiments of the present invention provide a deep reinforcement learning poisoning defense method and device based on the time series neural pathway. According to the learning characteristics of deep reinforcement learning, a time-series neural pathway for the deep reinforcement learning model is defined. The time-series neural pathway can correlate the input of the previou...
PUM
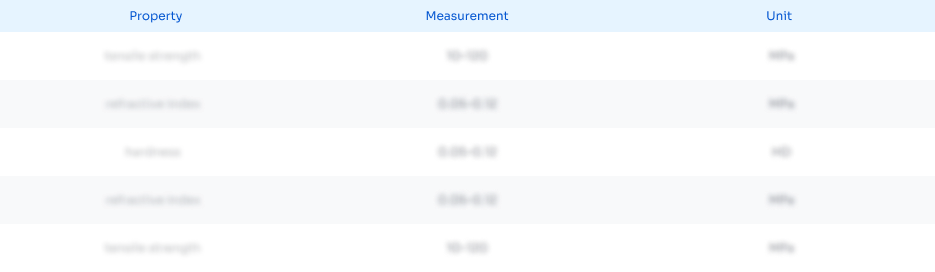
Abstract
Description
Claims
Application Information
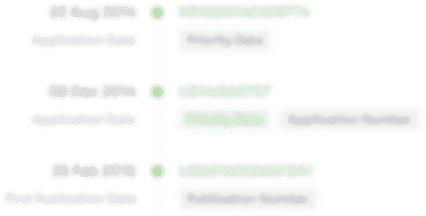
- R&D Engineer
- R&D Manager
- IP Professional
- Industry Leading Data Capabilities
- Powerful AI technology
- Patent DNA Extraction
Browse by: Latest US Patents, China's latest patents, Technical Efficacy Thesaurus, Application Domain, Technology Topic, Popular Technical Reports.
© 2024 PatSnap. All rights reserved.Legal|Privacy policy|Modern Slavery Act Transparency Statement|Sitemap|About US| Contact US: help@patsnap.com