Drug-target interaction prediction model method based on deep embedding learning of molecular graph and sequence
A prediction method and deep technology, applied in the field of deep embedding learning medicine, can solve problems such as limited training data, small covered space, limited model generalization ability, etc.
- Summary
- Abstract
- Description
- Claims
- Application Information
AI Technical Summary
Problems solved by technology
Method used
Image
Examples
Embodiment Construction
[0051] The following will clearly and completely describe the technical solutions in the embodiments of the present invention with reference to the accompanying drawings in the embodiments of the present invention. Obviously, the described embodiments are only some, not all, embodiments of the present invention. Based on the embodiments of the present invention, all other embodiments obtained by persons of ordinary skill in the art without making creative efforts belong to the protection scope of the present invention.
[0052] The present invention provides a drug-target interaction prediction method based on deep embedded learning of graphs and sequences as shown in the figure, which is characterized in that the graph representation of drug molecules is used as input, that is, the atoms of molecules are regarded as Vertices, bonds between atoms are regarded as edges, and text sequences composed of amino acid elements are used as the input of the protein processing model.
[...
PUM
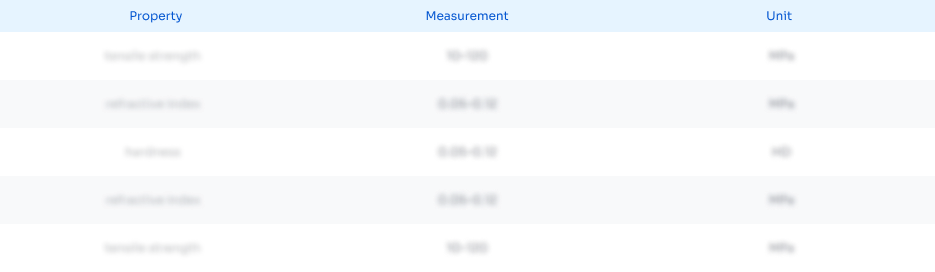
Abstract
Description
Claims
Application Information
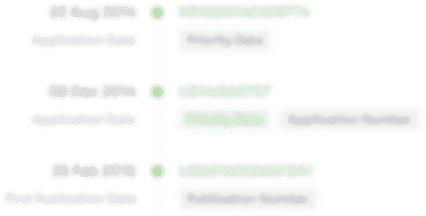
- R&D
- Intellectual Property
- Life Sciences
- Materials
- Tech Scout
- Unparalleled Data Quality
- Higher Quality Content
- 60% Fewer Hallucinations
Browse by: Latest US Patents, China's latest patents, Technical Efficacy Thesaurus, Application Domain, Technology Topic, Popular Technical Reports.
© 2025 PatSnap. All rights reserved.Legal|Privacy policy|Modern Slavery Act Transparency Statement|Sitemap|About US| Contact US: help@patsnap.com