Intrinsic decomposition method of hyperspectral image based on digital surface model
A technology of digital surface model and hyperspectral image, applied in the field of hyperspectral image eigendecomposition, can solve the problem of low eigendecomposition accuracy of hyperspectral image, achieve the effect of eliminating spectral information degradation and improving accuracy
- Summary
- Abstract
- Description
- Claims
- Application Information
AI Technical Summary
Problems solved by technology
Method used
Image
Examples
specific Embodiment approach 1
[0061] Specific implementation mode one: the following combination figure 1 Illustrate this embodiment, the hyperspectral image eigendecomposition method based on digital surface model assistance described in this embodiment, it comprises:
[0062] S1. Input hyperspectral images and digital surface model data, and calculate geometric components;
[0063] S2. Calculate and obtain a local prior matrix;
[0064] S3. Calculate and obtain a non-local prior matrix;
[0065] S4. Perform eigendecomposition according to the geometric component, local prior matrix and non-local prior matrix, and output hyperspectral reflectance and ambient light.
specific Embodiment approach 2
[0066] Specific implementation mode 2: This implementation mode further explains the implementation mode 1. The specific method for calculating and obtaining the geometric component described in S1 includes:
[0067] input hyperspectral image
[0068] Input digital surface model elevation data
[0069] Among them, h k =[h k (λ 1 ), h k (λ 2 ),…,h k (λ d )] T ,k=1,2,...,u represents the spectral feature of each pixel, k=1,2,...,u represents the index of each pixel, λ represents the wavelength, d represents the number of bands, u represents the height The number of spectral image pixels, z 1 ,z 2 ,…,z u Indicates the elevation corresponding to each pixel, represents the domain;
[0070] Calculate the normal of each pixel and obtain the normal feature:
[0071] in, Represents the projection of the normal on the x, y, and z space coordinate axes;
[0072] Calculate the geometric component J: J=[J 1 ,J 2 ,...,J u ] ú ;
[0073] in,
[0074]
[0075]...
specific Embodiment approach 3
[0078] Specific implementation mode three: this implementation mode further explains implementation mode two, and the specific method for calculating and obtaining the local prior matrix described in S2 includes:
[0079] Traverse the index k=1,2,...,u of each pixel and build a dictionary D k =[h 1 ,..., h k-1 ,h k+1 ,..., h u ,I d ];
[0080] Among them, I d Represents a d-dimensional identity matrix;
[0081] Calculate h according to the following formula k in dictionary D k The sparse representation coefficient α in :
[0082] min α ‖α‖ 1 subject to h k =D k α;
[0083] Each element W of the local prior matrix W kj Obtained by the following assignment:
[0084]
[0085] W kj Indicates the element of row k and column j of W;
[0086] alpha j Indicates the sparse representation coefficient for column j, α j-1 Indicates the sparse representation coefficients for the j-1th column.
PUM
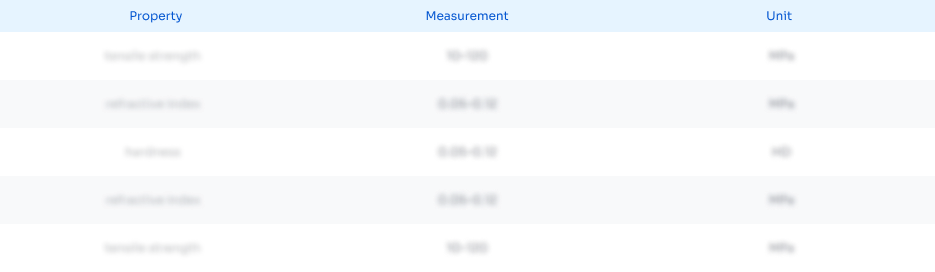
Abstract
Description
Claims
Application Information
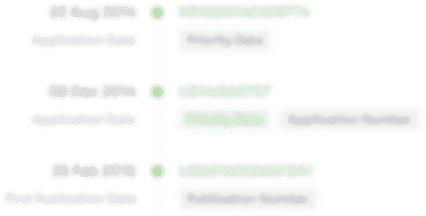
- R&D
- Intellectual Property
- Life Sciences
- Materials
- Tech Scout
- Unparalleled Data Quality
- Higher Quality Content
- 60% Fewer Hallucinations
Browse by: Latest US Patents, China's latest patents, Technical Efficacy Thesaurus, Application Domain, Technology Topic, Popular Technical Reports.
© 2025 PatSnap. All rights reserved.Legal|Privacy policy|Modern Slavery Act Transparency Statement|Sitemap|About US| Contact US: help@patsnap.com