Wind power prediction method based on improved depth extreme learning machine
An extreme learning machine, wind power prediction technology, applied in prediction, kernel method, biological model and other directions, can solve the problems of falling into local optimal solution, prone to over-fitting, narrow application range, etc., to speed up the convergence speed, The effect of improving quality and improving accuracy
- Summary
- Abstract
- Description
- Claims
- Application Information
AI Technical Summary
Problems solved by technology
Method used
Image
Examples
Embodiment Construction
[0038] The present invention will be described in further detail below in conjunction with the accompanying drawings.
[0039] The invention proposes a wind power prediction method based on an improved deep extreme learning machine, comprehensively considers the compound weighted objective function of MAE, MMAPE and RMSE, and compares the gray wolf algorithm improved by the mixed compound weighted objective function with the deep extreme learning machine combined. The optimal number of nodes and the optimal regularization parameter C of each hidden layer of the DELM model are obtained by using the gray wolf algorithm improved by the mixed compound weighted objective function to train and optimize the DELM parameters, and then by including each hidden layer The DELM model with the optimal number of nodes in the layer and the optimal regularization parameter C predicts the wind power. Such as figure 1 As shown, it specifically includes the following steps:
[0040] Step 1: Ob...
PUM
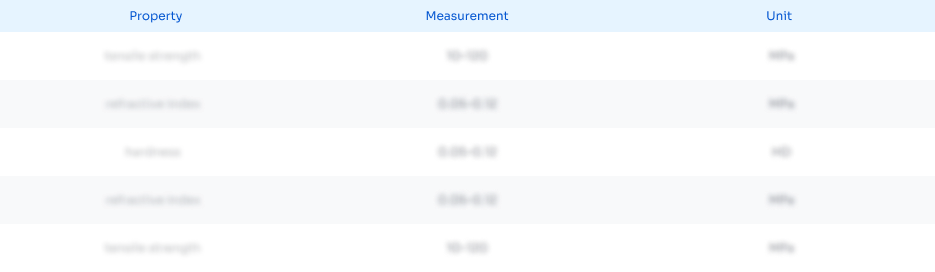
Abstract
Description
Claims
Application Information
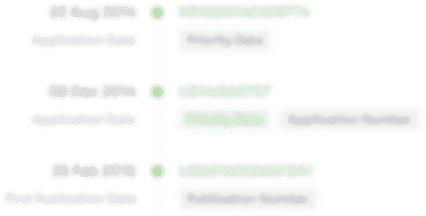
- R&D Engineer
- R&D Manager
- IP Professional
- Industry Leading Data Capabilities
- Powerful AI technology
- Patent DNA Extraction
Browse by: Latest US Patents, China's latest patents, Technical Efficacy Thesaurus, Application Domain, Technology Topic, Popular Technical Reports.
© 2024 PatSnap. All rights reserved.Legal|Privacy policy|Modern Slavery Act Transparency Statement|Sitemap|About US| Contact US: help@patsnap.com