Image classification method, training method of image classification model and equipment
A classification method and classification model technology, applied in neural learning methods, biological neural network models, character and pattern recognition, etc., can solve the problems of complex data, high cost of sample data labeling, and few data set labels, so as to improve the classification effect , good learning effect, pay attention to the effect of continuity
- Summary
- Abstract
- Description
- Claims
- Application Information
AI Technical Summary
Problems solved by technology
Method used
Image
Examples
Example Embodiment
[0058] Example one
[0059] This embodiment proposes an image classification method. figure 1 It is a flow chart of an image classification method according to an embodiment of the present invention. like figure 1 As shown, the method includes S10-S30.
[0060] S10: Separate the image to be classified into multiple patches, generate each PATCH corresponding to the PATCH vector; to reduce each PATCH vector through the linear layer, a plurality of downthetted PATCH vectors are spliced to obtain the first sequence vector; A variable vector is embedded in the first sequence vector, where the second sequence vector is obtained, wherein the variable vector is the same as that of each reduced PATCH vector size, and the variable vector corresponds to the more The PATCH that is mostly representative of the characteristics of the image to be classified.
[0061] Alternatively, the image to be classified is divided into a plurality of patches, and a sequence is paved, followed by a downtim...
Example Embodiment
[0087] Example 2
[0088] This embodiment provides a training method of an image classification model for training the image classification model constituted by the image classification method described in the first embodiment. figure 2 It is a flow chart of a training method for an image classification model provided by the embodiment of the present invention. like figure 2 As shown, the method includes steps S01-S06.
[0089] S01: Get a training data set D, where the training data set includes a label data set D. l And label data set D u Each training data is a training image, each with label data D l Label D l Real category Y l .
[0090] S02: For each label data d l Enhanced random data is enhanced, and there is a label data set after enhancement For each label Data D u Enhanced the random data of K, get K enhanced label data set K = 1, ..., k, all D u K And collect Put each label Data D u K The image classification model corresponding to the image classification method a...
Example Embodiment
[0125] Example three
[0126] Figure 4 It is a flow chart of another image classification method according to an embodiment of the present invention. This method implements the network learning process of image classification based on Transformer's semi-regulatory algorithm, including training phase and prediction stages. like Figure 4 As shown, the method includes S1-S8.
[0127] S1: Predict the pseudo label. First, all non-tagged data is enhanced, repeat K times, and then predict the enhanced label data input model, obtain K pseudo tags, and finally take an average operation as a pseudo-label without label data.
[0128] S2: Image block processing. The input image is cut into multiple patches, and a sequence is paved, followed by a reduced operation by the learning linear projection. Finally, the first sequence of sequences corresponding to all PATCH is embedded in a vector (referred to as "Patch Embed Retide Vector). This vector initialization is random, learning (ie, variable...
PUM
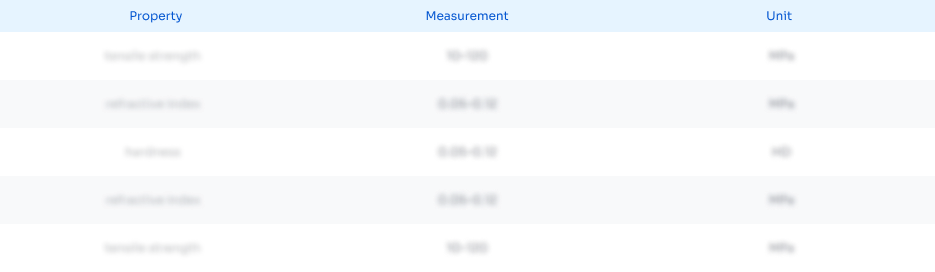
Abstract
Description
Claims
Application Information
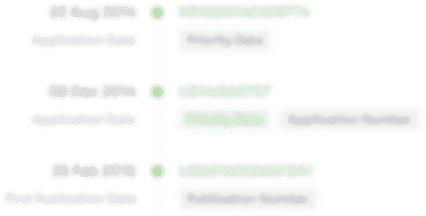
- R&D Engineer
- R&D Manager
- IP Professional
- Industry Leading Data Capabilities
- Powerful AI technology
- Patent DNA Extraction
Browse by: Latest US Patents, China's latest patents, Technical Efficacy Thesaurus, Application Domain, Technology Topic.
© 2024 PatSnap. All rights reserved.Legal|Privacy policy|Modern Slavery Act Transparency Statement|Sitemap