Human skeleton action recognition method based on difference graph convolutional neural network
A convolutional neural network and action recognition technology, applied in the field of robot learning and computer vision, can solve the problems of low recognition accuracy, weak robustness, and large amount of calculation, and achieve high recognition accuracy, improved learning ability, and high recognition accuracy Effect
- Summary
- Abstract
- Description
- Claims
- Application Information
AI Technical Summary
Problems solved by technology
Method used
Image
Examples
Embodiment Construction
[0032] The present invention will be described in detail below in conjunction with the accompanying drawings and specific embodiments. This embodiment is carried out on the premise of the technical solution of the present invention, and detailed implementation and specific operation process are given, but the protection scope of the present invention is not limited to the following embodiments.
[0033] This embodiment provides a human skeleton action recognition method based on a difference graph convolutional neural network. The training flow chart and network architecture diagram of the method are respectively figure 1 and figure 2 As shown, it specifically includes the following steps:
[0034] S1. Preprocess the bone data according to the general method, eliminate irrelevant bone data and incomplete repair data, and normalize the data of each dimension in the bone data to the [0,1] interval.
[0035] S2. Preliminarily design the difference graph convolutional neural ne...
PUM
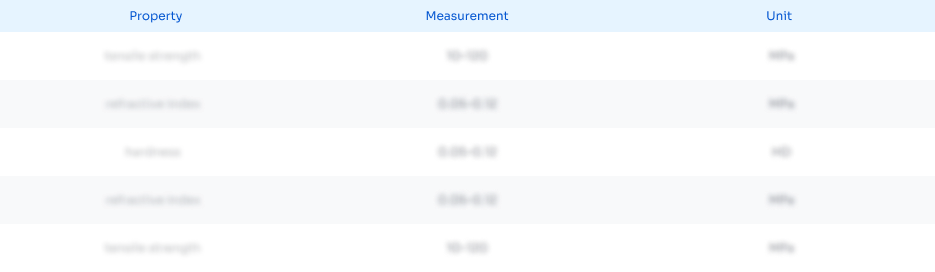
Abstract
Description
Claims
Application Information
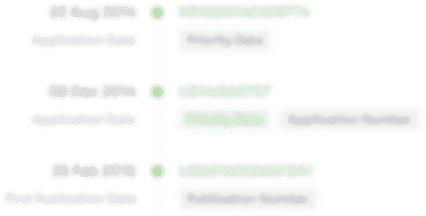
- R&D
- Intellectual Property
- Life Sciences
- Materials
- Tech Scout
- Unparalleled Data Quality
- Higher Quality Content
- 60% Fewer Hallucinations
Browse by: Latest US Patents, China's latest patents, Technical Efficacy Thesaurus, Application Domain, Technology Topic, Popular Technical Reports.
© 2025 PatSnap. All rights reserved.Legal|Privacy policy|Modern Slavery Act Transparency Statement|Sitemap|About US| Contact US: help@patsnap.com