Logging reservoir identification and prediction method based on machine learning
A technology of reservoir identification and machine learning, applied in the field of machine learning, can solve problems such as poor applicability of logging interpretation models, complex pore structure, and strong reservoir heterogeneity
- Summary
- Abstract
- Description
- Claims
- Application Information
AI Technical Summary
Problems solved by technology
Method used
Image
Examples
Embodiment 1
[0029] Embodiment 1: (taking 28 wells in identifying Li 8-Li 57 as an example)
[0030] A method for identifying and predicting well logging reservoirs based on machine learning, specifically including the following four steps:
[0031]Step S1: Prepare a large number of well logging data samples, build a well logging database, select conventional well logging data and gas test data as input, and mark the data, obtain the data information of existing wells according to the pre-feature selection rules, and select jing , ceng, DEPT, AC, PE, SP, CAL, GR, U, TH, K, AC, CNL, DEN, RLLS, and RLLD were used as characteristic factors, and 2544 pieces of logging data were obtained, including 738 pieces of gas layer, gas and water There are 549 layers, 125 poor gas layers, 747 gas-bearing water layers, 233 water layers, and 152 dry layers, and the data of each layer is marked with the task category number;
[0032] Step S2: According to the collected data set, preprocess the data and nor...
PUM
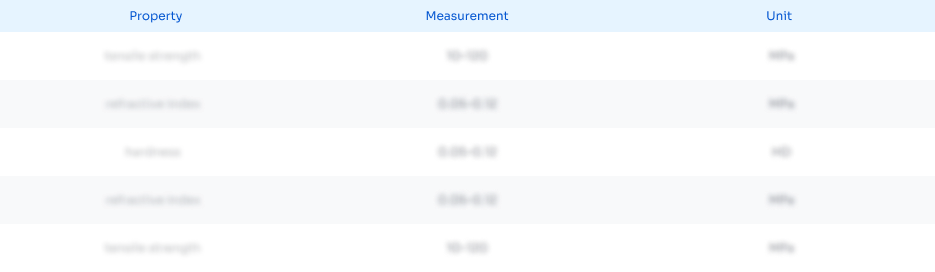
Abstract
Description
Claims
Application Information
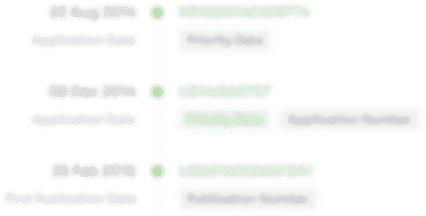
- R&D Engineer
- R&D Manager
- IP Professional
- Industry Leading Data Capabilities
- Powerful AI technology
- Patent DNA Extraction
Browse by: Latest US Patents, China's latest patents, Technical Efficacy Thesaurus, Application Domain, Technology Topic, Popular Technical Reports.
© 2024 PatSnap. All rights reserved.Legal|Privacy policy|Modern Slavery Act Transparency Statement|Sitemap|About US| Contact US: help@patsnap.com