Tumor tissue pathology classification system and method based on adaptive proportional learning
A tumor tissue and classification system technology, applied in image analysis, image data processing, instruments, etc., can solve the problems of less training constraints, difficult to cover pathological slices, time-consuming and labor-intensive manual reading, etc., to improve the recognition accuracy, The effect of expanding data utilization and improving data utilization
- Summary
- Abstract
- Description
- Claims
- Application Information
AI Technical Summary
Problems solved by technology
Method used
Image
Examples
Embodiment Construction
[0045] The specific embodiments of the present invention will be described in further detail below in conjunction with the accompanying drawings.
[0046] Such as figure 1 As shown, the present invention provides a tumor histopathological classification system based on adaptive ratio learning, which includes a ratio labeling module, a foreground segmentation and image block positioning module, and an adaptive multi-magnification integrated ratio learning module;
[0047] The proportional labeling module acquires several tumor tissue pathological slices and digitally scans them, manually labels the scanned pathological images according to the target category of the classification task, and constructs a data set; the specific process is as follows
[0048] The total dataset is recorded as , including a total of n full-size digital scan images of tumor tissue pathological sections obtained by hematoxylin-eosin (HE) staining (Whole Slide Image, WSI) ,Right now . The classi...
PUM
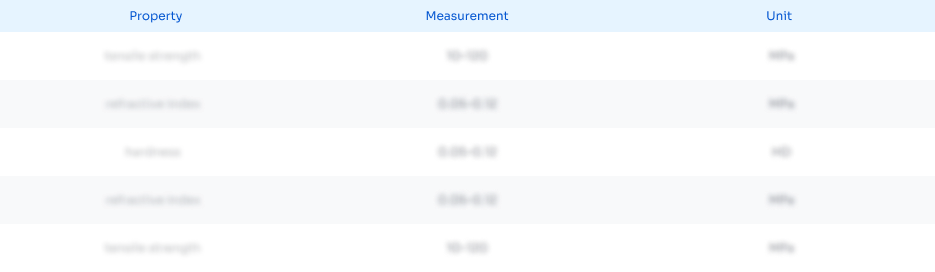
Abstract
Description
Claims
Application Information
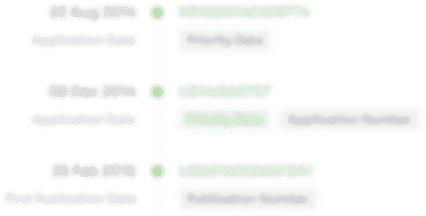
- R&D Engineer
- R&D Manager
- IP Professional
- Industry Leading Data Capabilities
- Powerful AI technology
- Patent DNA Extraction
Browse by: Latest US Patents, China's latest patents, Technical Efficacy Thesaurus, Application Domain, Technology Topic, Popular Technical Reports.
© 2024 PatSnap. All rights reserved.Legal|Privacy policy|Modern Slavery Act Transparency Statement|Sitemap|About US| Contact US: help@patsnap.com