Feature selection method and device based on conditional mutual information, equipment and storage medium
A feature selection method and conditional mutual information technology, applied in digital data information retrieval, computer parts, character and pattern recognition, etc., to achieve the effect of improving accuracy and efficiency
- Summary
- Abstract
- Description
- Claims
- Application Information
AI Technical Summary
Problems solved by technology
Method used
Image
Examples
Embodiment approach
[0049] see figure 1 As shown, the present invention provides a feature selection method based on conditional mutual information, comprising the following steps:
[0050] S1. Read the sample data, form a set of candidate features as a candidate feature set F; each sample has a corresponding classification, and take out the category features in the sample separately to form a category attribute C; initialize the feature set S to an empty set; Calculate the variance of each feature in the candidate feature set F, remove the features with zero variance in the candidate feature set F, and update the candidate feature set F. The sample data is the data generated by the calculation example. Suppose there are n samples, each sample has a physical quantity, and n samples of a certain physical quantity form a matrix with n rows and 1 column. This matrix is the eigenvector corresponding to this physical quantity, a The physical quantity constitutes a large matrix of n rows and a colum...
Embodiment 1
[0063] see figure 1 As shown, the present invention provides a feature selection method based on conditional mutual information, comprising the following steps:
[0064] S1. Read the data, form a set of candidate features as the candidate feature set F; initialize the feature set S as an empty set; calculate the variance of each feature in the candidate feature set F, and remove the features with zero variance in the candidate feature set F , and update the candidate feature set F.
[0065] S2. Calculate the mutual information between each candidate feature and category attribute C in the candidate feature set F updated in step S1, and put the candidate feature or empirical key feature with the largest mutual information into the feature set S as the initial selection feature, and select The candidate features of are deleted from the candidate feature set F; and the feature set S and the candidate feature set F are updated; the weight coefficient α is set, and the value range...
Embodiment 2
[0077] The present invention takes a test system standardized by IEEE10 machines and 39 nodes in a certain area as an example to carry out verification experiments; the simulation data of the 10 machines and 39 nodes power system is used as an input sample. Active power P, reactive power Q, node voltage amplitude V and node voltage phase angle θ have 170 candidate features. The category attribute C contains two categories, stable and unstable. A total of 3000 samples, including 1500 stable samples and 1500 unstable samples. During model training and prediction, the samples are randomly divided into 2000 training samples and 1000 prediction samples. In this experiment, the Relief algorithm and the mutual information selection algorithm were set as controls. All three algorithms selected 30 features to predict whether they were stable or not, and compared the accuracy. In order to reduce the error, each set of experiments was randomly divided into training set and test set 10 ...
PUM
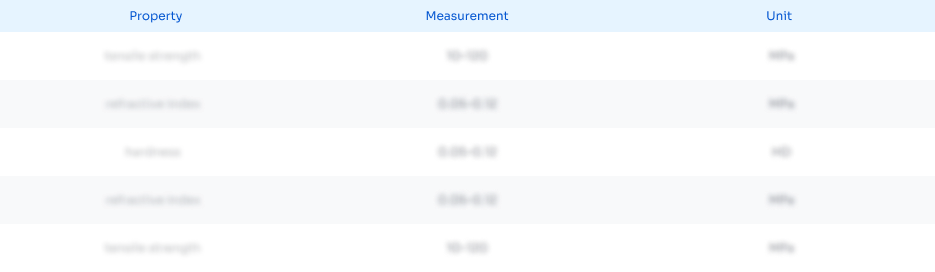
Abstract
Description
Claims
Application Information
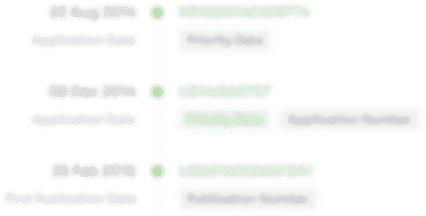
- Generate Ideas
- Intellectual Property
- Life Sciences
- Materials
- Tech Scout
- Unparalleled Data Quality
- Higher Quality Content
- 60% Fewer Hallucinations
Browse by: Latest US Patents, China's latest patents, Technical Efficacy Thesaurus, Application Domain, Technology Topic, Popular Technical Reports.
© 2025 PatSnap. All rights reserved.Legal|Privacy policy|Modern Slavery Act Transparency Statement|Sitemap|About US| Contact US: help@patsnap.com