VAE-based medical care federated learning framework determination method
A deterministic method and federated technology, applied in the field of computer science, can solve problems such as poor effect of the global model, high communication cost of the federated learning framework, and slow convergence of the global model
- Summary
- Abstract
- Description
- Claims
- Application Information
AI Technical Summary
Problems solved by technology
Method used
Image
Examples
Embodiment 1
[0058] This embodiment provides a determination method based on the VAE healthcare federated learning framework, which specifically includes the following steps:
[0059] Step 1: The medical institution obtains the initialized VAE model and initializes the anomaly detection model from the cloud server;
[0060] Step 2: Each medical institution trains and initializes the VAE model based on its own data set, and obtains the initial VAE model according to the selection of the data set fe Model and initialize VAE gen model; initialize the VAE fe The data set for model training includes all data, initialized VAE gen The dataset for model training includes sample data;
[0061] Step 3: The cloud server will obtain the initial VAE from each medical institution fe Model and initialize VAE gen The gradient of the model, after gradient aggregation; initialize the trained first generation VAE fe Model and first generation initialization VAE gen Models are distributed to all medica...
Embodiment 2
[0069] On the basis of Embodiment 1, the data set of the home gateway is acquired by the sensors in the wearable device, the sensors include angular velocity and acceleration sensors, and each sensor contains sequence information on the xyz three axes; The method of body data is to use Gramian Angular Field technology to convert it into two-dimensional image data.
[0070] Specifically, such as Figure 4 As shown, the left side is the original sequence data, and the right side is the 6-dimensional image data. The advantage of this processing method is that no matter how long the sequence data is, it can be unified into a 3D image of the same size, which is convenient for subsequent processing.
Embodiment 3
[0072] On the basis of Example 2, the VAE fe The function of the model is to extract low-dimensional features from the original high-dimensional data, and make the features obey the normal distribution; the VAE gen The function of the model is to generate more abnormal samples; the training process is as follows:
[0073] Step 41: The cloud server initializes the trained first generation VAE fe Model and first generation initialization VAE gen The model is distributed to all medical institutions participating in the training. The total number of medical institutions is k, and the data set owned by the kth medical institution is
[0074] Step 42: First Generation Initialize VAE fe The encoding end and decoding end of the model are denoted as E fe and D fe ; Initialize the VAE for the first generation gen The encoding end and decoding end of the model are denoted as E gen and D gen ;Each node needs to minimize the following two loss functions: this node refers to the m...
PUM
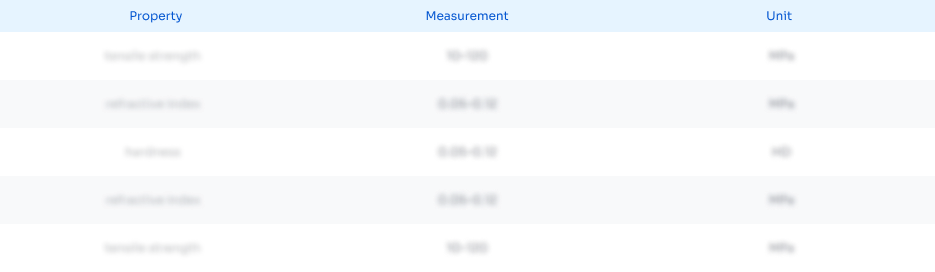
Abstract
Description
Claims
Application Information
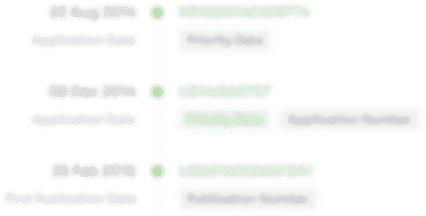
- R&D
- Intellectual Property
- Life Sciences
- Materials
- Tech Scout
- Unparalleled Data Quality
- Higher Quality Content
- 60% Fewer Hallucinations
Browse by: Latest US Patents, China's latest patents, Technical Efficacy Thesaurus, Application Domain, Technology Topic, Popular Technical Reports.
© 2025 PatSnap. All rights reserved.Legal|Privacy policy|Modern Slavery Act Transparency Statement|Sitemap|About US| Contact US: help@patsnap.com