Lightweight federated learning privacy protection method based on decentralized security aggregation
A security aggregation and decentralization technology, which is applied in machine learning, digital data protection, computer security devices, etc., can solve the problems of participants who are unable to afford secret sharing calculations, communication cost global model privacy leakage, etc., to reduce computing overhead, High availability, the effect of avoiding data privacy leakage
- Summary
- Abstract
- Description
- Claims
- Application Information
AI Technical Summary
Problems solved by technology
Method used
Image
Examples
Embodiment 1
[0062] This embodiment establishes a cooperation model based on a lightweight privacy-preserving federated learning method based on decentralized security aggregation in the present invention, such as figure 1 shown.
[0063] figure 1 The following decentralized secure aggregation scenario is described: each user holds a local dataset and updates the local model in the FL process. Each user randomly connects multiple edge nodes, the user divides the model parameters, generates carefully constructed global random numbers, and divides the global random numbers through parameter division. Send the split parameters and global nonce to the connected node. Edge nodes provide users with secure decentralized partial model aggregation, receive the divided partial models and perform partial model aggregation. Upload part of the aggregation model to the blockchain ledger for global aggregation, and get a global model covered by global random numbers. The blockchain ledger serves as a...
Embodiment 2
[0084] This embodiment compares the results of the method of the present invention in various scenarios, and verifies that the privacy protection method of the present invention has high training accuracy and efficiency. Compare this instance with existing methods that all aim to preserve data privacy during federated learning. Federated Learning has no privacy protections. HEDL uses HE encryption to protect the privacy of local models in distributed deep learning. DPFed guarantees the privacy of common global models unknown to users by adding DP noise in the global model. PSA and VerifyNet preserve the privacy of local models by overlaying random perturbations. These existing methods are compared with this method to obtain the comparison results of the accuracy and time cost of the training model, as shown in Table 4 and Table 5.
[0085] Table 4 Comparison results of precise accuracy of different methods under different user scales
[0086]
[0087] Table 5 Comparison...
Embodiment 3
[0093] In this embodiment, the results of the method of the present invention are compared in various scenarios, and it is verified that the privacy protection method of the present invention has a function of resisting inference attacks from members. Use member inference attack method to attack five different methods of federated learning, HEDL, DPFed, VerifyNet, PSA, using CIFAR-10 data set (https: / / www.cs.toronto.edu / ~kriz / cifar.html ) data set is used for membership inference attack, and the attack comparison results are shown in Table 6.
[0094] Table 6 Comparison results of different methods against membership inference attacks
[0095]
[0096]From Table 6, we can see that traditional FL, VerifyNet and PSA cannot defend against membership inference attacks, because the central server can still expose the global model. In HEDL, the server can only get the encrypted local model and global model, and the attack precision is very low. In this method, the attack accura...
PUM
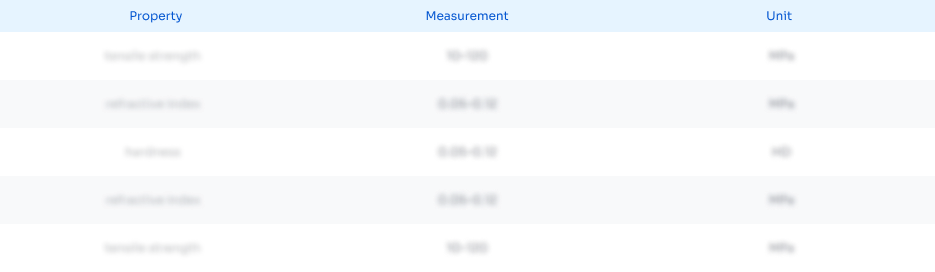
Abstract
Description
Claims
Application Information
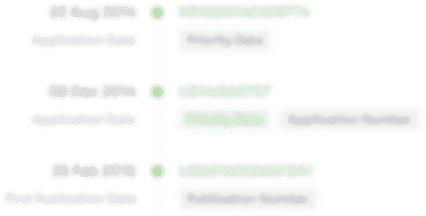
- R&D
- Intellectual Property
- Life Sciences
- Materials
- Tech Scout
- Unparalleled Data Quality
- Higher Quality Content
- 60% Fewer Hallucinations
Browse by: Latest US Patents, China's latest patents, Technical Efficacy Thesaurus, Application Domain, Technology Topic, Popular Technical Reports.
© 2025 PatSnap. All rights reserved.Legal|Privacy policy|Modern Slavery Act Transparency Statement|Sitemap|About US| Contact US: help@patsnap.com