Equipment fault diagnosis method based on improved 1DCNN-BiLSTM
A diagnostic method, a technology for equipment failure, applied in neural learning methods, testing of mechanical components, recognition of patterns in signals, etc., to achieve the effect of reducing interference
- Summary
- Abstract
- Description
- Claims
- Application Information
AI Technical Summary
Problems solved by technology
Method used
Image
Examples
Embodiment Construction
[0075] The present invention will be further described in detail below in conjunction with the accompanying drawings and specific embodiments.
[0076] The present invention uses industrial mechanical equipment fault diagnosis as the carrier, and uses LSTM and CNN algorithms as the main algorithm framework, and its model is as follows: figure 1 shown. The feature extraction module designs 1DCNN-BiLSTM dual-model channels, which are BiLSTM single-model channel and 1DCNN single-model channel. Among them, Channel1 is the Stacked Bi-LSTM neural network model, adjust the number of memory units of the BiLSTM network and the number of layers of the neural network, and realize the extraction of signal features of different dimensions; Channel4 is set as a 1DCNN model, and adjust the number of convolution kernels to realize different scales of signals Feature extraction; Channel2 and Channel3 add the improved SENet module on the basis of Channel1 and Channel4 respectively to perform ...
PUM
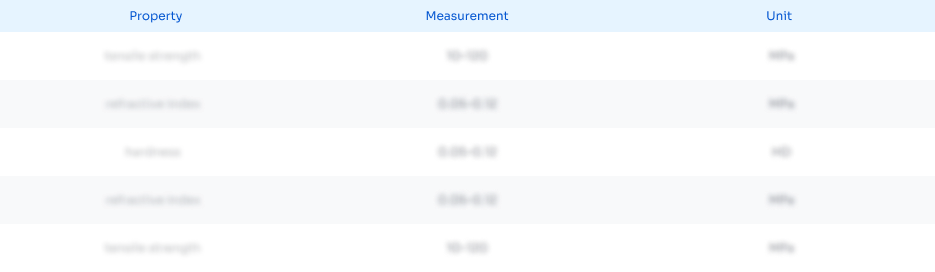
Abstract
Description
Claims
Application Information
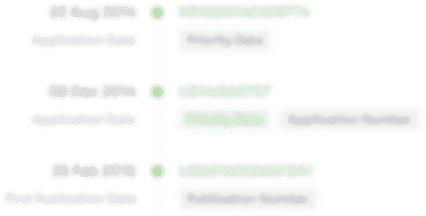
- R&D
- Intellectual Property
- Life Sciences
- Materials
- Tech Scout
- Unparalleled Data Quality
- Higher Quality Content
- 60% Fewer Hallucinations
Browse by: Latest US Patents, China's latest patents, Technical Efficacy Thesaurus, Application Domain, Technology Topic, Popular Technical Reports.
© 2025 PatSnap. All rights reserved.Legal|Privacy policy|Modern Slavery Act Transparency Statement|Sitemap|About US| Contact US: help@patsnap.com