Industrial defect detection method based on multi-task learning
A multi-task learning and defect detection technology, applied in the field of industrial defect detection, can solve the problems of low image contrast, large defect scale and appearance changes, unstable detection effect, etc., to achieve improved performance, good classification accuracy and generalization performance Effect
- Summary
- Abstract
- Description
- Claims
- Application Information
AI Technical Summary
Problems solved by technology
Method used
Image
Examples
Embodiment Construction
[0036] In order to verify the effect of the method provided in this embodiment, an experiment is done on the industrial data of photovoltaic cells in this embodiment. The data set contains ok pictures and pictures of 5 defect categories. The defect categories are fragments, cross hidden cracks, single hidden cracks, virtual welds and broken grids. The data set is divided into training set and test set according to the data ratio of 4:1. , the number of pictures in each category in the test set is shown in Table 1. Example pictures of each category such as Figure 4 As shown, it can be seen from the figure that there are mainly the following difficulties in processing the experimental data: the background of the picture is relatively complex, and the background texture will interfere with the judgment of defects; and the characteristics of some defects are similar, and the intra-class differences of some samples will be different. greater than the between-class differences. I...
PUM
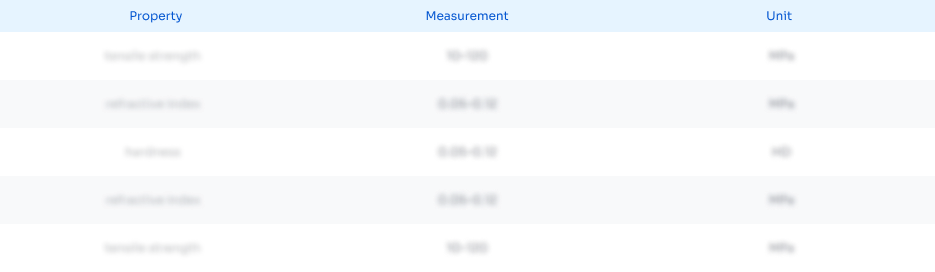
Abstract
Description
Claims
Application Information
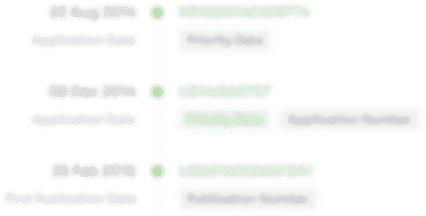
- R&D Engineer
- R&D Manager
- IP Professional
- Industry Leading Data Capabilities
- Powerful AI technology
- Patent DNA Extraction
Browse by: Latest US Patents, China's latest patents, Technical Efficacy Thesaurus, Application Domain, Technology Topic, Popular Technical Reports.
© 2024 PatSnap. All rights reserved.Legal|Privacy policy|Modern Slavery Act Transparency Statement|Sitemap|About US| Contact US: help@patsnap.com