Compound-target protein binding prediction method
A prediction method and compound technology, which is applied in the field of pharmaceutical research and development, can solve the problems of numerous adjusted parameters, high false positives, and low success rate, and achieve the effect of reducing intervention, reducing high false positives, and reducing false positives in prediction
- Summary
- Abstract
- Description
- Claims
- Application Information
AI Technical Summary
Problems solved by technology
Method used
Image
Examples
Embodiment 1
[0089] The "Recurrent Neural Network (Positive)" model in series with the "Recurrent Neural Network (Negative)" model predicts the compound-target protein binding relationship and system.
[0090] Schematic diagram of the overall system structure: see the attached Figure 3-4 .
[0091] System operating environment:
[0092] Hardware: CPU+GPU;
[0093] Software: Windows or Linux, Python+Tensorflow, PyTorch, Keras, etc.
[0094] Technical solutions:
[0095] as attached Figure 3-4 shown
[0096](1) Obtain compound-target protein binding data (positive sample), compound-target protein non-binding data (negative sample)
[0097] Compound-target protein binding data (positive sample), compound-target protein non-binding data (negative sample). The positive samples are the compound-target protein bindings that humans have discovered in scientific research activities, usually selected from ChemSpider, PubChem, BindingDB, ZINC, ChEMBL, etc.
[0098] Negative samples construc...
Embodiment 2
[0138] "Recurrent neural network (positive)" in series with "fully connected neural network (negative)" deep learning model to predict compound-target protein binding relationship and system.
[0139] Schematic diagram of the overall system structure: see the attached Figure 5 ,
[0140] System operating environment:
[0141] Hardware: CPU+GPU;
[0142]Software: Windows or Linux, Python+Tensorflow, PyTorch, Keras, etc.
[0143] (1) Obtain compound-target protein binding / unbinding data (positive sample / negative sample)
[0144] Same as the corresponding part of "Example 1".
[0145] (2-1) Extract training, testing and verification compound-target protein binding / unbinding data sets (positive sample / negative sample set)
[0146] With (2-1) part of " embodiment 1 " technical scheme.
[0147] (2-2) Construction of compound-target protein binding / non-binding deep learning model (positive / negative)
[0148] (i) Construct compound-target protein binding deep learning model (p...
Embodiment 3
[0176] "Recurrent neural network (positive)" in series with "convolutional neural network (negative)" deep learning model to predict compound-target protein binding relationship and system.
[0177] Schematic diagram of the overall system structure: see the attached Figure 6
[0178] System operating environment:
[0179] Hardware: CPU+GPU;
[0180] Software: Windows or Linux, Python+Tensorflow, PyTorch, Keras, etc.
[0181] (1) Obtain compound-target protein binding / unbinding data (positive sample / negative sample)
[0182] The part corresponding to "Example 1".
[0183] (2-1) Extract training, testing and verification compound-target protein binding / unbinding data sets (positive sample / negative sample set)
[0184] With (2-1) part of " embodiment 1 " technical scheme.
[0185] (2-2) Construction of compound-target protein binding / non-binding deep learning model (positive / negative model)
[0186] (i) Construct compound-target protein binding deep learning model (positi...
PUM
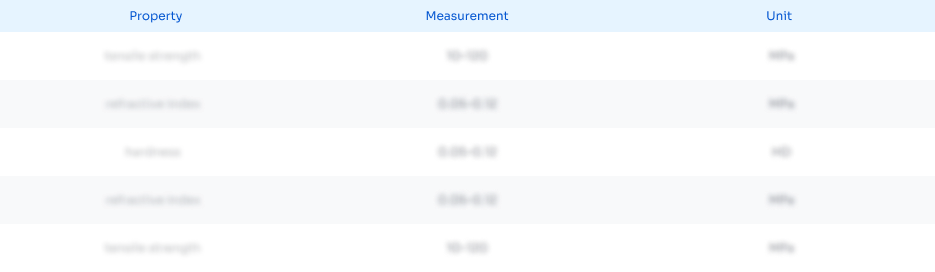
Abstract
Description
Claims
Application Information
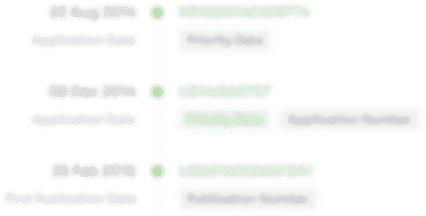
- R&D
- Intellectual Property
- Life Sciences
- Materials
- Tech Scout
- Unparalleled Data Quality
- Higher Quality Content
- 60% Fewer Hallucinations
Browse by: Latest US Patents, China's latest patents, Technical Efficacy Thesaurus, Application Domain, Technology Topic, Popular Technical Reports.
© 2025 PatSnap. All rights reserved.Legal|Privacy policy|Modern Slavery Act Transparency Statement|Sitemap|About US| Contact US: help@patsnap.com