Metalearning-based small sample Wi-Fi camouflage attack detection method and system
An attack detection, small sample technology, applied in the field of IoT security, can solve problems such as difficult application, GAN mode collapse, sample retraining, etc., to achieve the effect of meeting resource constraints and real-time requirements
- Summary
- Abstract
- Description
- Claims
- Application Information
AI Technical Summary
Problems solved by technology
Method used
Image
Examples
Embodiment Construction
[0056] In order to make the technical scheme and design ideas of the present invention clearer, the present invention will be further described in detail below in conjunction with the accompanying drawings.
[0057] A small-sample Wi-Fi spoofing attack detection method based on meta-learning, by constructing multiple auxiliary networks, learning meta-knowledge from different WAID historical tasks separately, and quickly transferring meta-knowledge to new WAID tasks.
[0058] The backbone network in the present invention is composed of CNN with 4 Blocks and 3 FC layers, where we define a convolution block as Block={Conv2d, Relu, BN, Max_pool}.
[0059] refer to figure 1 , figure 2 , a small-sample Wi-Fi forgery attack detection method based on meta-learning, including the following steps:
[0060] 1) Data preprocessing: The data comes from the publicly available AWID dataset, which contains the largest amount of Wi-Fi network traffic data collected from real network environm...
PUM
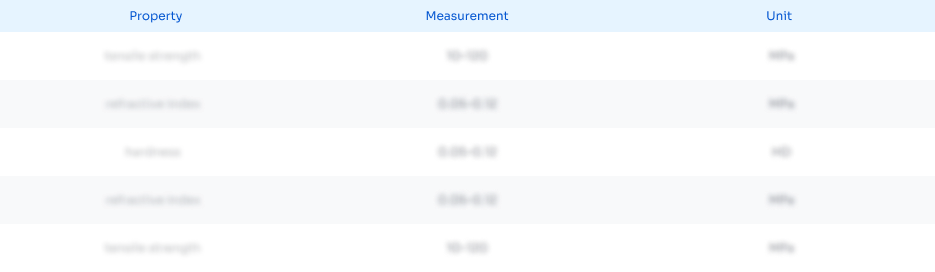
Abstract
Description
Claims
Application Information
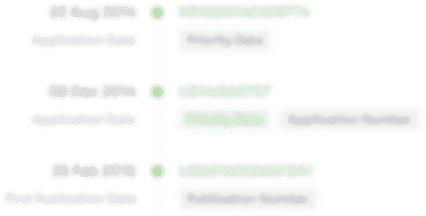
- R&D
- Intellectual Property
- Life Sciences
- Materials
- Tech Scout
- Unparalleled Data Quality
- Higher Quality Content
- 60% Fewer Hallucinations
Browse by: Latest US Patents, China's latest patents, Technical Efficacy Thesaurus, Application Domain, Technology Topic, Popular Technical Reports.
© 2025 PatSnap. All rights reserved.Legal|Privacy policy|Modern Slavery Act Transparency Statement|Sitemap|About US| Contact US: help@patsnap.com