Swin-Transform image denoising method and system based on channel attention
An attention and image technology, applied in the field of image processing, can solve the problems of easy loss of input noise image details, high computational memory and time consumption, and achieve the effect of overcoming high computational memory and time consumption
- Summary
- Abstract
- Description
- Claims
- Application Information
AI Technical Summary
Problems solved by technology
Method used
Image
Examples
Embodiment 1
[0041] The present embodiment provides a Swin-Transformer image denoising method based on channel attention, comprising the following steps:
[0042] Step S1: Obtain the original high-definition image data set, preprocess the original high-definition image data set, and obtain multiple pairs of training data sets of noisy images and high-definition images for the denoising network model.
[0043] Specifically, the present invention is trained on 800 training images of the image denoising training data set DIV2K, and tested on the public benchmark data sets BSD68 and Set12 of image denoising. DIV2K is a high-quality (2K resolution) image dataset consisting of 800 training images, 100 validation images and 100 test images. Set12 has 12 different scene noise images, and BSD68 has 68 different natural scene noise images.
[0044] Add Gaussian noise to 800 high-definition images in DIV2K, and generate 800 pairs of noise / clear images as the original training set D. Convert all ima...
Embodiment 2
[0071] Based on the same inventive concept, this embodiment provides a Swin-Transformer image denoising system based on channel attention, the problem-solving principle of which is similar to the xx method described above, and repeated descriptions will not be repeated.
[0072] This embodiment provides a Swin-Transformer image denoising system based on channel attention, including:
[0073] The data preparation module is used to obtain the original high-definition image data set, preprocess the original high-definition image data set, and obtain a training data set of noisy images and high-definition images for denoising network model training;
[0074] The shallow feature extraction module is used to input the noisy image to the shallow feature extraction network in the denoising network model to extract feature information to obtain a shallow feature map;
[0075] The deep feature extraction module is used to use the shallow feature map as the input of the deep feature extr...
PUM
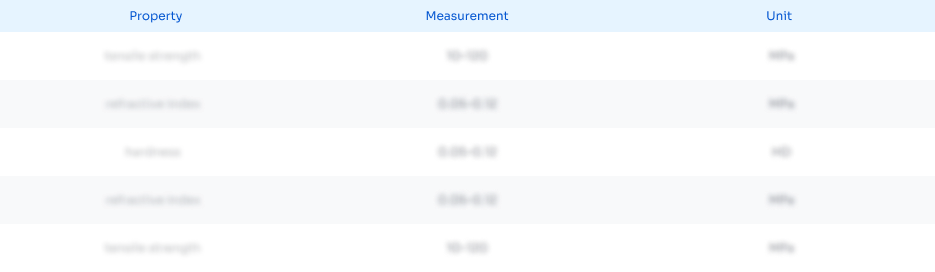
Abstract
Description
Claims
Application Information
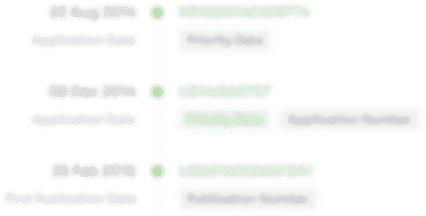
- Generate Ideas
- Intellectual Property
- Life Sciences
- Materials
- Tech Scout
- Unparalleled Data Quality
- Higher Quality Content
- 60% Fewer Hallucinations
Browse by: Latest US Patents, China's latest patents, Technical Efficacy Thesaurus, Application Domain, Technology Topic, Popular Technical Reports.
© 2025 PatSnap. All rights reserved.Legal|Privacy policy|Modern Slavery Act Transparency Statement|Sitemap|About US| Contact US: help@patsnap.com