Indoor 3D positioning method based on generalized regression neural network
A generalized regression and neural network technology, applied in the field of indoor 3D positioning based on generalized regression neural network, can solve the problems of inflexible coordination between global and local search capabilities, optimization capabilities and efficiency to be improved, and low positioning accuracy. Achieve the effect of solving over-fitting phenomenon, solving poor prediction effect and high positioning accuracy
- Summary
- Abstract
- Description
- Claims
- Application Information
AI Technical Summary
Problems solved by technology
Method used
Image
Examples
Embodiment 1
[0100] Refer Figure 1 - Figure 16 The present invention provides an indoor 3D positioning method based on a generalized regression neural network, including the following steps:
[0101] Step 1: Get the intensity indication value of the receiving signal of the test site, determine the range of the test site according to the intensity indication value.
[0102] Step 2: Collect the three-dimensional coordinate sample data of the test nodes in the test site, train and establish a generalized regression neural network model.
[0103] Step 3: Optimize the gray wolf algorithm, the improved gray wolf optimization algorithm IGWO adjusts the flow chart of smoothing factor Image 6 As shown, the smooth factor is adjusted using the optimized gray wolf algorithm to obtain the step of optimal optimization smooth factor σ, including:
[0104] Step 301: Using the good point set method to initialize the gray wolf population of gray wolf algorithm, obtain the steps of initial group distribution of ...
Embodiment 2
[0149] In the traditional gray wolf algorithm, the initial wolf population is randomly generated, the random distribution of population makes the initial individual cannot traverse the entire search space, causing the loss of better solution, may also fall into local optimal value, lowered The optimization ability of the algorithm. The initial population having a uniform distribution can improve the optimization effect of the algorithm to some extent. The present invention adopts a combination of a combination method to initialize the gray wolf population to obtain a more uniform and stable initial population distribution.
[0150] The number of initial groups in the wolf group is N. The dimension of the search space is D.
[0151] rim d = E d 1 ≤ D ≤ D (25)
[0152] The Dimension of the Item of the Centralized Point Point is expressed as:
[0153]
[0154] Put the best point to the initial population of the gray wolf, the search space is 1 dimension, then the value of the 1-dim...
Embodiment 3
[0162] The experiment is selected in a 12m × 10m office ring, based on the ZigBee positioning system, prepare 5 Corporate Nodes (APs), and placed in four corners and center positions of the test field ceiling, the data used to receive the positioning node There are various obstacles in the test site. The test area is divided into 120 small areas, each small area size of 1 m × 1m, and the center position of the small area is used as the test point. The tester carries the positioning node with you, from the (0,0) position, traversing each test small area. At each test point, the positioning node sends 100 packets to the 5 trick nodes, and forms [RSSI 1 , RSSI 2 , RSSI 3 , RSSI 4 , RSSI 5 (x i Y i ,z i )] Sample data, i = 1, 2, 3, ..., 120, as sample set data. 100 sets of data from 120 sets of sample data as a training sample set, and the remaining 20 groups are set as a test sample set. The RSSI value of the training sample set is used as the input of the network model. Training the...
PUM
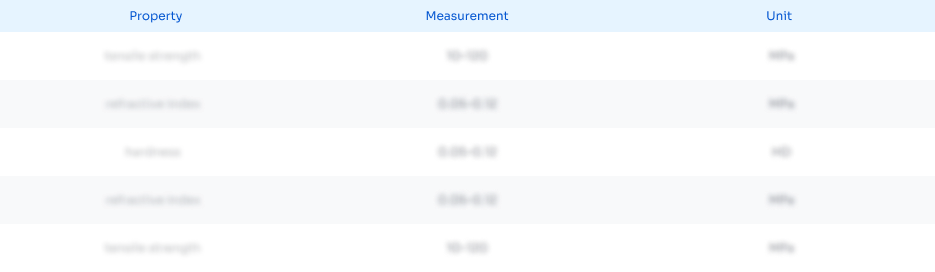
Abstract
Description
Claims
Application Information
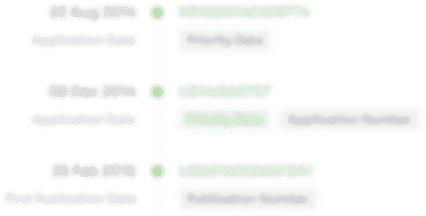
- R&D Engineer
- R&D Manager
- IP Professional
- Industry Leading Data Capabilities
- Powerful AI technology
- Patent DNA Extraction
Browse by: Latest US Patents, China's latest patents, Technical Efficacy Thesaurus, Application Domain, Technology Topic.
© 2024 PatSnap. All rights reserved.Legal|Privacy policy|Modern Slavery Act Transparency Statement|Sitemap