Capsule network image positioning improved algorithm based on CNN
A network image and positioning algorithm technology, applied in the application field of deep neural network technology, can solve problems such as over-fitting and convolutional neural network sample quantity dependence, and achieve the effect of improving accuracy and reducing dependence.
- Summary
- Abstract
- Description
- Claims
- Application Information
AI Technical Summary
Problems solved by technology
Method used
Image
Examples
Embodiment Construction
[0044] Step 1: Perform image enhancement and noise reduction on the input to reduce the impact on features.
[0045] Step 2: Improve the xception model, add a downsampling layer, a batch normalization layer, and a Dropout layer. The Dropout layer parameter is set to 0.5 to ensure that half of the neurons are discarded for each incoming data.
[0046] Step 3: Pass the training set into the xception model for feature extraction
[0047] Step 4: Pass the extracted features into the capsule network, and perform image position feature extraction again.
[0048] Step 5: Output the positioning coordinates and mark them in the imported image.
[0049] The above-mentioned content is only a preferred embodiment of the present invention, but the scope of protection of the present invention is not limited thereto. Any person familiar with the technical field can make some improvements without departing from the present invention. and retouching should be covered within the protection sc...
PUM
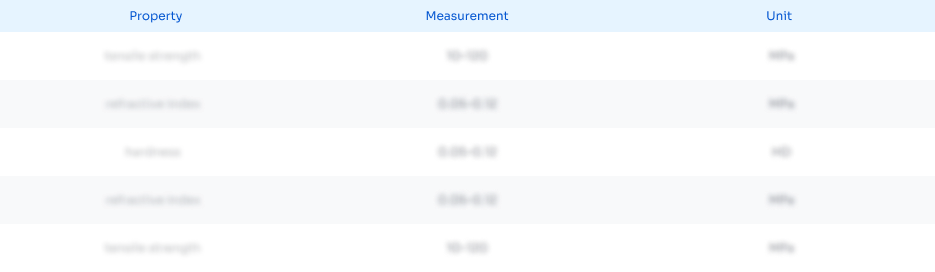
Abstract
Description
Claims
Application Information
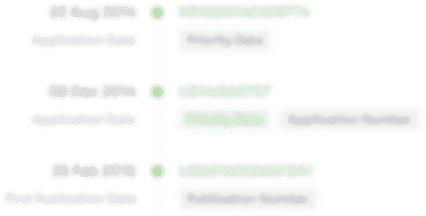
- R&D
- Intellectual Property
- Life Sciences
- Materials
- Tech Scout
- Unparalleled Data Quality
- Higher Quality Content
- 60% Fewer Hallucinations
Browse by: Latest US Patents, China's latest patents, Technical Efficacy Thesaurus, Application Domain, Technology Topic, Popular Technical Reports.
© 2025 PatSnap. All rights reserved.Legal|Privacy policy|Modern Slavery Act Transparency Statement|Sitemap|About US| Contact US: help@patsnap.com