Three-dimensional point cloud semantic segmentation method and system based on dynamic aggregation
A 3D point cloud and semantic segmentation technology, applied in the field of image processing, can solve the problems of limited feature extraction ability of multi-layer perceptron, limited ability of spatial semantic information, unstable semantic feature weight change, etc., to achieve rich feature expression ability, Maintain model performance and avoid the effects of deconvolution operations
- Summary
- Abstract
- Description
- Claims
- Application Information
AI Technical Summary
Problems solved by technology
Method used
Image
Examples
Embodiment Construction
[0052] The following will clearly and completely describe the technical solutions in the embodiments of the present invention with reference to the drawings in the embodiments of the present invention. Obviously, the described embodiments are part of the embodiments of the present invention, not all of them. Based on the embodiments of the present invention, all other embodiments obtained by persons of ordinary skill in the art without making creative efforts shall fall within the protection scope of the present invention.
[0053] The method examples of the present invention are given below.
[0054] A certain self-driving car is equipped with a laser radar, and the laser radar is used to collect point cloud data, and the corresponding points are marked, and the semantic segmentation method of the three-dimensional point cloud based on dynamic aggregation of the present invention is used for semantic segmentation. combine Figure 1 ~ Figure 2 , the method of the present inve...
PUM
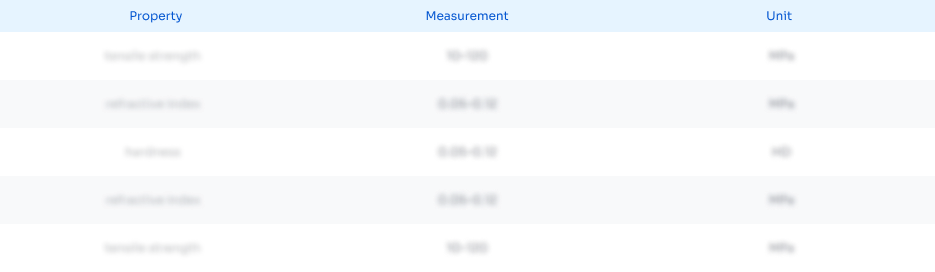
Abstract
Description
Claims
Application Information
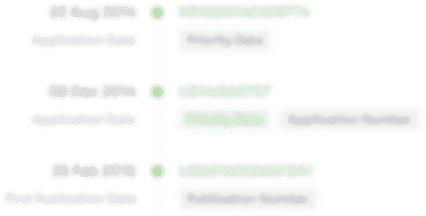
- Generate Ideas
- Intellectual Property
- Life Sciences
- Materials
- Tech Scout
- Unparalleled Data Quality
- Higher Quality Content
- 60% Fewer Hallucinations
Browse by: Latest US Patents, China's latest patents, Technical Efficacy Thesaurus, Application Domain, Technology Topic, Popular Technical Reports.
© 2025 PatSnap. All rights reserved.Legal|Privacy policy|Modern Slavery Act Transparency Statement|Sitemap|About US| Contact US: help@patsnap.com