Joint training method based on multi-party 3D printing database
A 3D printing and database technology, applied in electrical digital data processing, instruments, design optimization/simulation, etc., can solve problems such as application and development limitations, and achieve the effects of easy convergence, reduced transmission volume, reduced demand and input costs
- Summary
- Abstract
- Description
- Claims
- Application Information
AI Technical Summary
Problems solved by technology
Method used
Image
Examples
Embodiment 1
[0034] A method for joint training based on multi-party 3D printing databases, including multiple training members and servers, the model of the training members is wi , the data for each training member is Xi , , labeled as y i ; the server's model is W , and the server's model W Model with training members wi The network structure is the same; including the following steps:
[0035] Step S100: at j During training iterations, the training members i read Xi one of batch The data bi , and model wi The forward propagation of to get the predicted label , and then according to the actual label y i , the calculated model wi The loss function, and then use the backpropagation algorithm to get the gradient matrix G i ;
[0036] Step S200: train members i pair gradient matrix G i The elements in are sorted from large to small according to the absolute value, and the first m elements are selected to obtain the corresponding sparse matrix , the filling elem...
Embodiment 2
[0040] This embodiment is optimized on the basis of Embodiment 1. Before the iterative training, the model is initialized: the server to the model W Initialize and distribute the initialization results to all training members, and the model W i Perform initialization to determine the gradient upload scale coefficient α, attenuation coefficient ρ, and learning rate γ.
[0041] Further, in the step S200, the model is obtained statistically W i The total number of elements in is M , calculate the number of gradient elements that need to be uploaded this time .
[0042] Other parts of this embodiment are the same as those of Embodiment 1, so details are not repeated here.
Embodiment 3
[0044] This embodiment is optimized on the basis of Embodiment 1 or 2, and the correlation calculation in the step S300 is as follows:
[0045]
[0046] in:
[0047]
[0048] in: D KL for KL Divergence,
[0049] P Indicates the quality of each training member's own data,
[0050] Q Indicates the data quality of all samples of the server.
[0051] Other parts of this embodiment are the same as those of Embodiment 1 or 2 above, so details are not repeated here.
PUM
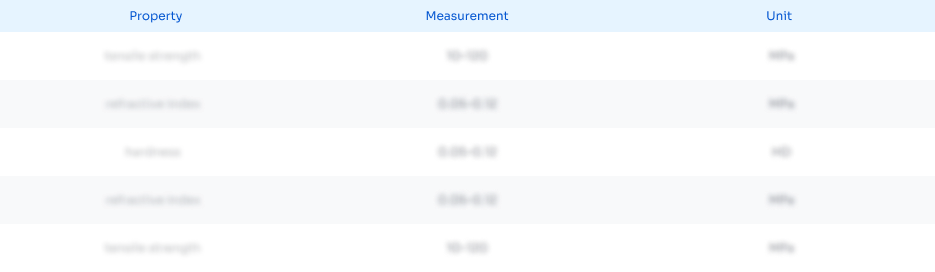
Abstract
Description
Claims
Application Information
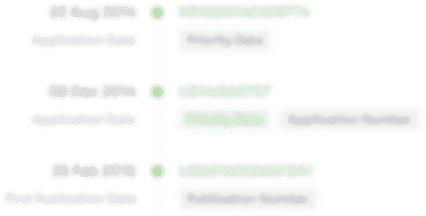
- Generate Ideas
- Intellectual Property
- Life Sciences
- Materials
- Tech Scout
- Unparalleled Data Quality
- Higher Quality Content
- 60% Fewer Hallucinations
Browse by: Latest US Patents, China's latest patents, Technical Efficacy Thesaurus, Application Domain, Technology Topic, Popular Technical Reports.
© 2025 PatSnap. All rights reserved.Legal|Privacy policy|Modern Slavery Act Transparency Statement|Sitemap|About US| Contact US: help@patsnap.com