Neural network prediction method based on boiler combustion characteristics
A boiler combustion and neural network technology, applied in the field of neural network prediction based on boiler combustion characteristics, can solve problems such as too large initial population range, long convergence time of genetic algorithm, etc., to overcome the defects of network initial connection weight and threshold, and guarantee Group Diversity, Guaranteed Convergence Effects
- Summary
- Abstract
- Description
- Claims
- Application Information
AI Technical Summary
Problems solved by technology
Method used
Image
Examples
Embodiment 1
[0039] At present, the BP neural network is mostly used to establish the boiler combustion prediction model, and the output of the BP neural network is comprehensively optimized based on the genetic algorithm to find the best boiler efficiency setting value and the best NO x The emission set point, by adjusting the input of BP neural network, makes the boiler efficiency and NO x Emissions are optimized. However, in the above method, due to the use of a genetic algorithm for optimization, there is a problem that the initial population range is too large, resulting in a long time for the convergence of the genetic algorithm. Therefore, in order to solve the above problems, such as figure 1 and figure 2 As shown, the present embodiment provides a neural network prediction method based on boiler combustion characteristics, which includes the following steps:
[0040] S1. The gas volume and flue gas oxygen content are used as the input of the BP neural network, and the boiler e...
Embodiment 2
[0062] Since the BP neural network algorithm is a method of adjusting connection weights and thresholds based on gradient descent, the initial connection weights and thresholds of the network structure are randomly set before training. Once the initial parameter setting is unreasonable, it will easily fall into In the local minimum defect. Genetic Algorithm (GA) has the ability of global search, and is not easily restricted by local optimum in the search process. Combining the large-scale nonlinear mapping capability of the BP neural network algorithm with the global optimization characteristics of the GA algorithm can overcome the defects of the traditional BP neural network algorithm that randomly generates network initial connection weights and thresholds, and improve the learning rate of the BP neural network algorithm. and linear approximation capabilities. Therefore, on the basis of Embodiment 1, this embodiment provides a genetic algorithm-based optimization of the ini...
PUM
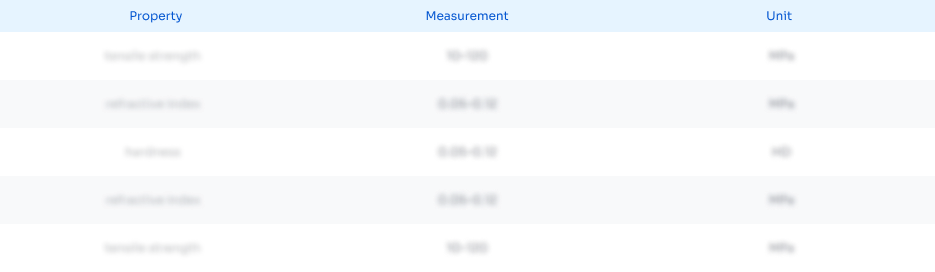
Abstract
Description
Claims
Application Information
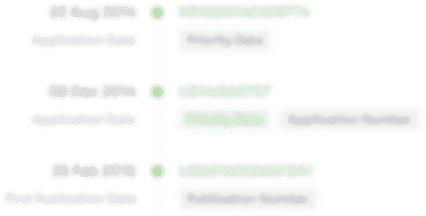
- R&D Engineer
- R&D Manager
- IP Professional
- Industry Leading Data Capabilities
- Powerful AI technology
- Patent DNA Extraction
Browse by: Latest US Patents, China's latest patents, Technical Efficacy Thesaurus, Application Domain, Technology Topic, Popular Technical Reports.
© 2024 PatSnap. All rights reserved.Legal|Privacy policy|Modern Slavery Act Transparency Statement|Sitemap|About US| Contact US: help@patsnap.com