Model training method and system, nonvolatile storage medium and computer terminal
A technology of model training and training data sets, applied in the field of machine learning, can solve the problem of high consumption of GPU computing resources, achieve the effect of improving convergence speed and training efficiency
- Summary
- Abstract
- Description
- Claims
- Application Information
AI Technical Summary
Problems solved by technology
Method used
Image
Examples
Embodiment 1
[0032] The purpose of unsupervised learning or self-supervised learning is to learn models or features with strong expressive ability through unlabeled data. Usually, it is necessary to define a pretext task to guide the training of the model. Agents include, but are not limited to, change prediction, image completion, spatial or temporal sequence prediction, clustering, data generation, etc. Among them, the method based on contrastive learning adopts a dual-branch network, and the input of the network is usually two data enhancements of images. Its purpose is to make the two data enhancements of the same image closer in feature space, while the corresponding feature distances of the data enhancements of different images are longer. The contrast learning method needs more negative samples, needs a large batch size, or needs a storage queue to store historical feature vectors, but even so, the diversity of negative samples is still relatively scarce.
[0033] However, the method of...
Embodiment 2
[0059] The embodiment of the application also provides a model training method, which is applied to a GPU cluster, wherein the GPU cluster includes a plurality of GPUs, and each GPU of the plurality of GPUs has a first feature extraction network and a first full connection layer, wherein the network structures of the first feature extraction networks in the plurality of GPUs are the same, and the first full connection layer is cut based on the second full connection layer in the target neural network model; It should be noted that the alternative implementation scheme of the GPU cluster in this embodiment can adopt the implementation scheme of the GPU cluster described in Embodiment 1, but it is not limited to this. such as Figure 3 As shown, the method includes:
[0060] S302, a first GPU of a plurality of GPUs initializes a first feature extraction network of the first GPU, and extracts a first sample feature in a target training data set by using the initialized first feature e...
Embodiment 3
[0073] The embodiment of the application provides a method embodiment of a model training method. It should be noted that the steps shown in the flowchart of the figure can be executed in a computer system such as a set of computer-executable instructions, and although the logical sequence is shown in the flowchart, in some cases, the steps shown or described can be executed in a sequence different from that here.
[0074] The method embodiment provided by the embodiment of the application can be executed in a mobile terminal, a computer terminal or a similar computing device. Figure 4 A block diagram of the hardware structure of a computer terminal (or mobile device) used to implement the model training method is shown. such as Figure 4 As shown, the computer terminal 40 (or mobile device 40) may include one or more (402a, 402b, ..., 402n are shown in the figure) processors 402 (the processors 402 may include, but are not limited to, processing devices such as microprocessor MCU ...
PUM
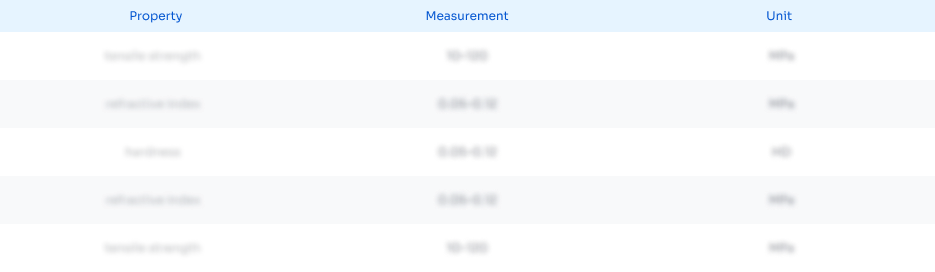
Abstract
Description
Claims
Application Information
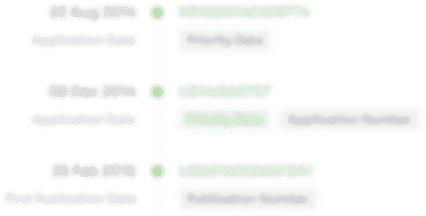
- R&D Engineer
- R&D Manager
- IP Professional
- Industry Leading Data Capabilities
- Powerful AI technology
- Patent DNA Extraction
Browse by: Latest US Patents, China's latest patents, Technical Efficacy Thesaurus, Application Domain, Technology Topic, Popular Technical Reports.
© 2024 PatSnap. All rights reserved.Legal|Privacy policy|Modern Slavery Act Transparency Statement|Sitemap|About US| Contact US: help@patsnap.com