Train bearing fault diagnosis method based on improved generative adversarial network
A technology of fault diagnosis and network, applied in the direction of biological neural network model, neural learning method, instrument, etc., can solve the problems of failure classification and design of fault diagnosis model, etc., and achieve the goal of improving diagnosis recognition rate and quality Effect
- Summary
- Abstract
- Description
- Claims
- Application Information
AI Technical Summary
Problems solved by technology
Method used
Image
Examples
experiment example
[0053] Adopt the method that above-mentioned embodiment provides, utilize rail transit transmission fault simulation comprehensive experiment platform to collect train bearing signal data set as training set, sampling frequency is set to 25kHz in the experiment, motor output speed is set to 1200rpm, and loading force is set to 3000N; The data set includes cage minor faults (CI), inner ring minor faults (II), rolling element minor faults (RI), cage severe faults (CS), inner ring severe faults (IS) and rolling element severe faults (RS) ; Among them, the number of samples in the CI category is 5000, while the number of samples in other fault categories is only 100, and the train bearing data is seriously unbalanced; the relationship between the number of iterations and the loss value is as follows Figure 5 As shown, among them, Figure 5 a is the discriminative loss, Figure 5 b is the generation loss, Figure 5 c is the classification loss. After 250 times of training, the l...
PUM
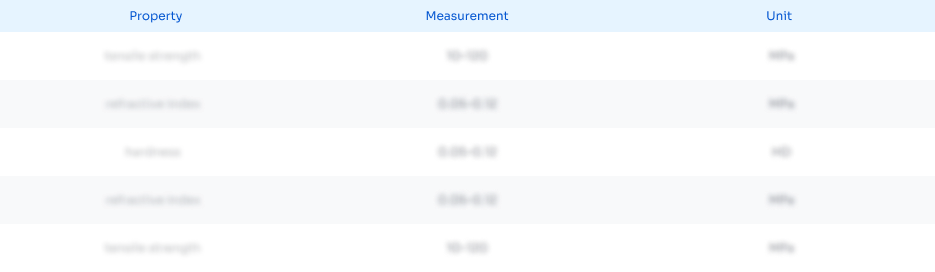
Abstract
Description
Claims
Application Information
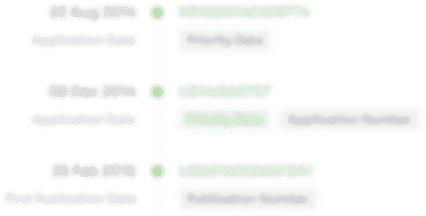
- R&D Engineer
- R&D Manager
- IP Professional
- Industry Leading Data Capabilities
- Powerful AI technology
- Patent DNA Extraction
Browse by: Latest US Patents, China's latest patents, Technical Efficacy Thesaurus, Application Domain, Technology Topic, Popular Technical Reports.
© 2024 PatSnap. All rights reserved.Legal|Privacy policy|Modern Slavery Act Transparency Statement|Sitemap|About US| Contact US: help@patsnap.com