Bone action recognition method based on learnable PL-GCN and ECLSTM
A PL-GCN and action recognition technology, applied in the field of action recognition, can solve the problems that the spatial features of bone joint points cannot be fully extracted, and the importance is not considered.
- Summary
- Abstract
- Description
- Claims
- Application Information
AI Technical Summary
Problems solved by technology
Method used
Image
Examples
Embodiment Construction
[0086] The technical solutions in the embodiments of the present invention will be described clearly and in detail below with reference to the accompanying drawings in the embodiments of the present invention. The described embodiments are only some of the embodiments of the invention.
[0087] The technical scheme that the present invention solves the above-mentioned technical problems is:
[0088] This patent proposes a spatiotemporal graph convolutional skeleton action recognition method with learning ability. The overall framework of the model is as follows figure 1 shown.
[0089] First, in order to fully obtain the spatial and temporal features of action video samples, the present invention adopts a two-stream network framework, and proposes a graph convolutional network with self-learning ability to extract the spatial feature relationship between skeleton joint points. To fully extract , we consider stacking 9 layers of learnable graph convolutional network modules t...
PUM
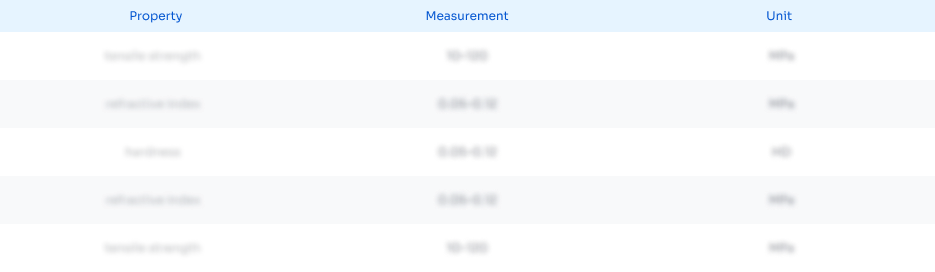
Abstract
Description
Claims
Application Information
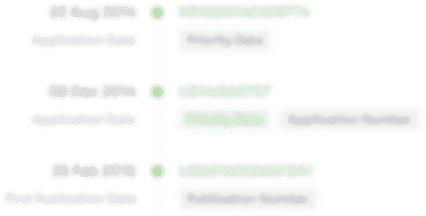
- R&D Engineer
- R&D Manager
- IP Professional
- Industry Leading Data Capabilities
- Powerful AI technology
- Patent DNA Extraction
Browse by: Latest US Patents, China's latest patents, Technical Efficacy Thesaurus, Application Domain, Technology Topic, Popular Technical Reports.
© 2024 PatSnap. All rights reserved.Legal|Privacy policy|Modern Slavery Act Transparency Statement|Sitemap|About US| Contact US: help@patsnap.com