Zero-carbon building optimization design method based on deep reinforcement learning
A technology of optimization design and reinforcement learning, applied in the field of zero-carbon buildings, it can solve the problems of high degree of visualization, too subjective decision-making process of icon-based decision-making method, and failure of comprehensive evaluation of target value, so as to improve computing efficiency and high generalization ability. , reducing the effect of overfitting problems
- Summary
- Abstract
- Description
- Claims
- Application Information
AI Technical Summary
Problems solved by technology
Method used
Image
Examples
Embodiment Construction
[0069] In order to make the purposes, technical solutions and advantages of the embodiments of the present invention clearer, the technical solutions in the embodiments of the present invention will be clearly and completely described below with reference to the accompanying drawings in the embodiments of the present invention. Obviously, the described embodiments These are some embodiments of the present invention, but not all embodiments. Based on the embodiments of the present invention, all other embodiments obtained by those of ordinary skill in the art without creative work fall within the protection scope of the present invention.
[0070] The following is a detailed description of the design of a zero-carbon office building in a cold area:
[0071] as attached figure 1 , a zero-carbon building optimization design method based on deep reinforcement learning provided by an embodiment of the present invention includes the following steps:
[0072] Step 1: Construction o...
PUM
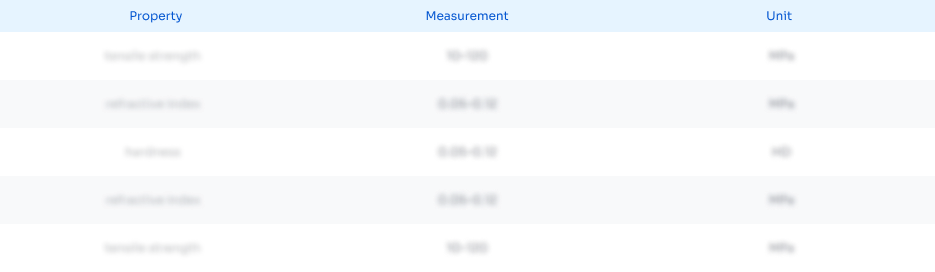
Abstract
Description
Claims
Application Information
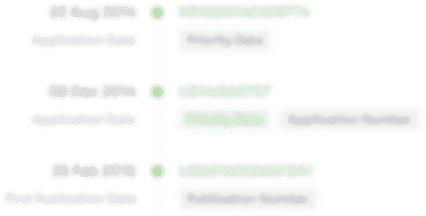
- R&D Engineer
- R&D Manager
- IP Professional
- Industry Leading Data Capabilities
- Powerful AI technology
- Patent DNA Extraction
Browse by: Latest US Patents, China's latest patents, Technical Efficacy Thesaurus, Application Domain, Technology Topic, Popular Technical Reports.
© 2024 PatSnap. All rights reserved.Legal|Privacy policy|Modern Slavery Act Transparency Statement|Sitemap|About US| Contact US: help@patsnap.com