Federal learning load prediction method based on dynamic weighted aggregation
A load forecasting and dynamic weighting technology, applied in neural learning methods, forecasting, ensemble learning, etc., can solve the problems of reduced accuracy of aggregated models, inconsistent data quality, uneven training effects of local models, etc., to speed up convergence, improve The effect of convergence speed
- Summary
- Abstract
- Description
- Claims
- Application Information
AI Technical Summary
Problems solved by technology
Method used
Image
Examples
Embodiment Construction
[0025] Aiming at the problem that the existing federated learning load forecasting methods do not fully evaluate the pros and cons of local models when aggregating global models, a federated learning load forecasting method based on dynamic weighted aggregation is proposed. The edge computing device uses local data to train the neural network, and the obtained network parameter change vector is uploaded to the cloud server, and the pairwise similarity calculation is performed to generate a similarity matrix. Using the similarity matrix, calculate the consistency vector between the local models in this round of training. Based on the consistency between the accuracy of each round of local models on the server-side validation set and the local models, the network parameter changes of different local models are weighted to achieve the effect of local model cleaning, and the cloud server will download the updated global model. Send it to the edge computing device, and repeat the a...
PUM
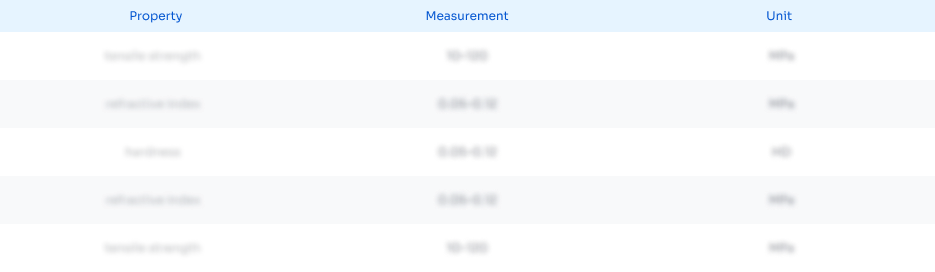
Abstract
Description
Claims
Application Information
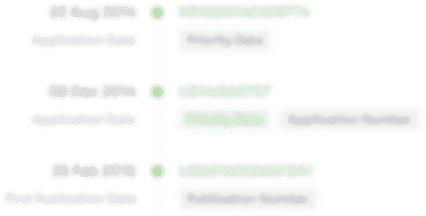
- Generate Ideas
- Intellectual Property
- Life Sciences
- Materials
- Tech Scout
- Unparalleled Data Quality
- Higher Quality Content
- 60% Fewer Hallucinations
Browse by: Latest US Patents, China's latest patents, Technical Efficacy Thesaurus, Application Domain, Technology Topic, Popular Technical Reports.
© 2025 PatSnap. All rights reserved.Legal|Privacy policy|Modern Slavery Act Transparency Statement|Sitemap|About US| Contact US: help@patsnap.com