Regulable load prediction method and system for users participating in orderly power utilization
A load forecasting and electricity user technology, which is applied in the field of adjustable load forecasting of users participating in orderly electricity consumption and load forecasting of electricity user regulation and control, can solve the difficulty of differentiated dispatching, the different sensitivity of grid dispatching response, and the difficulty in dispatching effect. Guarantee and other issues to achieve the effect of ensuring reliable power supply and ensuring safe and stable operation
- Summary
- Abstract
- Description
- Claims
- Application Information
AI Technical Summary
Problems solved by technology
Method used
Image
Examples
Embodiment 1
[0064] like figure 1 As shown, the controllable load prediction method for participating in orderly electricity users in this embodiment includes:
[0065] 1) Construct the power characteristic index vector based on user power data and grid load data;
[0066] 2) Input the power characteristic index vector into the pre-trained machine learning model to obtain the corresponding user participation control load.
[0067] The power characteristic index vector constructed in step 1) of this embodiment includes: power consumption fluctuation rate, power consumption peak-to-valley difference, peak-to-average power consumption ratio, staggered peak load difference, weekly rest load difference, weekly load drop rate, maintenance load There are 8 characteristic indicators of difference and maximum utilization hour rate. User power data and grid load data refer to the hourly power data of the user in one month and the hourly load data of the power grid in the province within a month. Th...
Embodiment 2
[0096] This embodiment is basically the same as the first embodiment, and the main difference is: in order to improve the accuracy of the machine learning model in this embodiment, the function expression of the machine learning model in step 2) of this embodiment is:
[0097]
[0098] In the above formula, f(φ(X)) represents the output of the machine learning model, n is the total number of training samples, α i , is a pair of Lagrange multipliers, K(φ(X), φ(X i )) is the kernel function, φ(X) represents the input test group power characteristic index vector, φ(X) i ) represents the input training group i-th power characteristic index vector, and b is the displacement.
[0099] Kernel function K(φ(X), φ(X i )) can select a kernel function that satisfies the MERCER condition as required, for example, as an optional implementation, in this embodiment, the kernel function K(φ(X), φ(X) i )) adopts the radial basis function RBF, and its function expression is:
[0100] ...
PUM
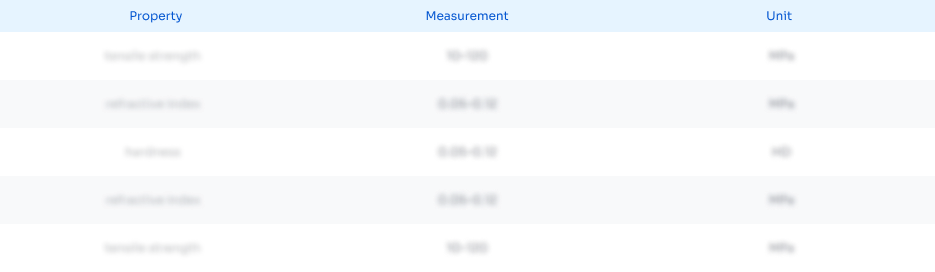
Abstract
Description
Claims
Application Information
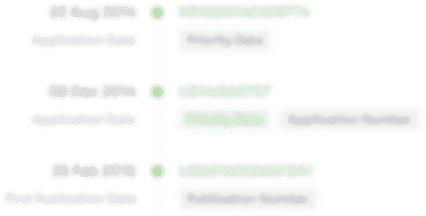
- R&D
- Intellectual Property
- Life Sciences
- Materials
- Tech Scout
- Unparalleled Data Quality
- Higher Quality Content
- 60% Fewer Hallucinations
Browse by: Latest US Patents, China's latest patents, Technical Efficacy Thesaurus, Application Domain, Technology Topic, Popular Technical Reports.
© 2025 PatSnap. All rights reserved.Legal|Privacy policy|Modern Slavery Act Transparency Statement|Sitemap|About US| Contact US: help@patsnap.com