Night image defogging algorithm based on deep learning
A deep learning and imaging technology, applied in neural learning methods, computing, image enhancement, etc., can solve problems such as difficult foggy image restoration
- Summary
- Abstract
- Description
- Claims
- Application Information
AI Technical Summary
Problems solved by technology
Method used
Image
Examples
Embodiment Construction
[0030] In order to make the objectives, technical solutions and advantages of the present invention clearer, the present invention will be further described in detail below with reference to the embodiments. It should be understood that the specific embodiments described herein are only used to explain the present invention, but not to limit the present invention.
[0031] A deep learning-based night image dehazing algorithm provided by an embodiment of the present invention includes the following steps:
[0032] Step 1: collect clear image data without fog at night, then perform scene depth ratio estimation on the image data, and perform fog processing on the clear image without fog at night based on the depth ratio map to establish a night "fog-free fog" image data set;
[0033] Step 2: First design the encoder part of the overall network. The foggy image at night is subjected to three dual-tree complex wavelet transform groups in the encoder to iteratively extract multi-sca...
PUM
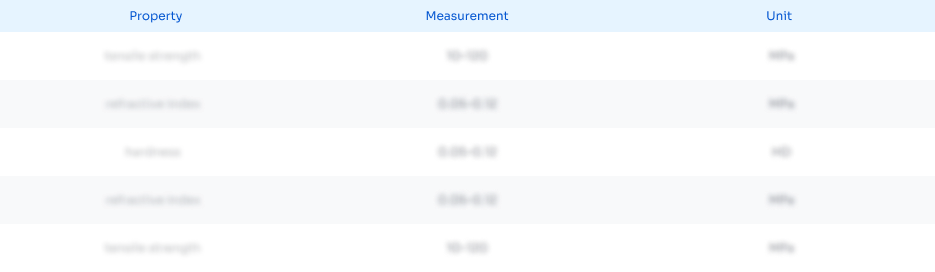
Abstract
Description
Claims
Application Information
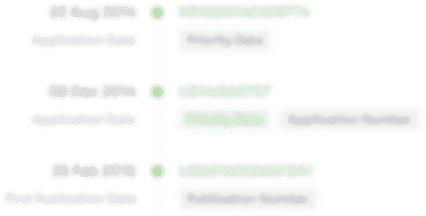
- R&D
- Intellectual Property
- Life Sciences
- Materials
- Tech Scout
- Unparalleled Data Quality
- Higher Quality Content
- 60% Fewer Hallucinations
Browse by: Latest US Patents, China's latest patents, Technical Efficacy Thesaurus, Application Domain, Technology Topic, Popular Technical Reports.
© 2025 PatSnap. All rights reserved.Legal|Privacy policy|Modern Slavery Act Transparency Statement|Sitemap|About US| Contact US: help@patsnap.com