Feature dispersion degree-based attention mechanism method and system
A technology of discreteness and attention, applied in the field of image processing, can solve the problems of losing the importance of features, weakening the importance of features, affecting the redistribution of feature map weights, etc., to achieve the effect of optimizing channel weight distribution and improving performance
- Summary
- Abstract
- Description
- Claims
- Application Information
AI Technical Summary
Problems solved by technology
Method used
Image
Examples
Embodiment Construction
[0041] The present application will be further described in detail below with reference to the accompanying drawings.
[0042] In the embodiments of the present application, in order to optimize the weight distribution of the neural network to the feature map and improve the performance of the neural network in classification and detection, an attention mechanism method and system based on the discrete degree of features are specifically disclosed. refer to figure 1 , is a flow chart of the steps of the attention mechanism method based on the degree of feature discreteness, including:
[0043] S10: Based on the initial feature map, obtain the target variance corresponding to the pixel value in the initial feature map;
[0044] S20: Based on the target variance and the sigmoid activation function, obtain the target weight value;
[0045] S30: Obtain a target feature map based on the target weight value and the initial feature map;
[0046] Among them, in the channel attentio...
PUM
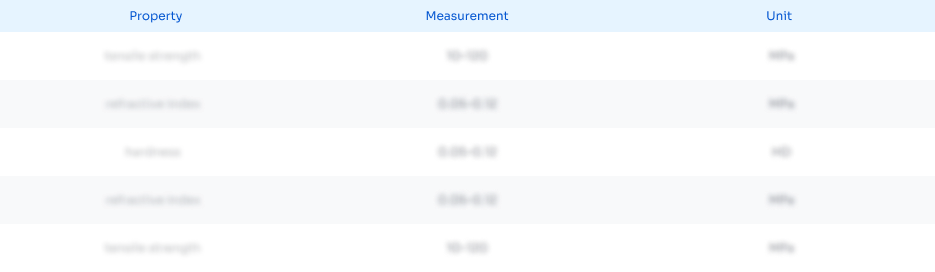
Abstract
Description
Claims
Application Information
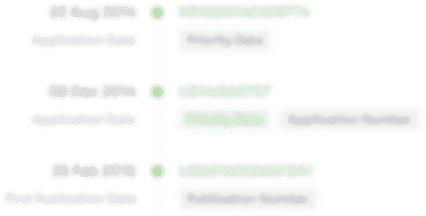
- R&D
- Intellectual Property
- Life Sciences
- Materials
- Tech Scout
- Unparalleled Data Quality
- Higher Quality Content
- 60% Fewer Hallucinations
Browse by: Latest US Patents, China's latest patents, Technical Efficacy Thesaurus, Application Domain, Technology Topic, Popular Technical Reports.
© 2025 PatSnap. All rights reserved.Legal|Privacy policy|Modern Slavery Act Transparency Statement|Sitemap|About US| Contact US: help@patsnap.com