Method and apparatus for learning to classify patterns and assess the value of decisions
A technique for determining values and patterns, applied in the field of learning strategies, which can solve problems such as the inability of differential learning to provide guarantees, the limitation of mathematical characteristics of neural network representative models, and the lack of mention.
- Summary
- Abstract
- Description
- Claims
- Application Information
AI Technical Summary
Problems solved by technology
Method used
Image
Examples
Embodiment Construction
[0034] refer to figure 1 , illustrates a system 20 that includes a stochastically parameterized neural network classification / value estimation model 21 of concepts to be learned. The neural network defining the model 21 may be any self-learning model that can be taught or trained to perform the classification or value estimation task represented by the mathematical mapping defined by the network. For the purposes of this application, the term "neural network" includes any mathematical model whose set of parameters constitutes a differentiable (as defined in calculus) mathematical mapping from a numerical input pattern to a set of output numbers, each corresponding to a A unique classification of the input pattern or an estimate of the value of a unique decision made in response to the input pattern. The neural network model can take many implementation forms. For example, it can be simulated in the form of software running on a general-purpose digital computer. It can be im...
PUM
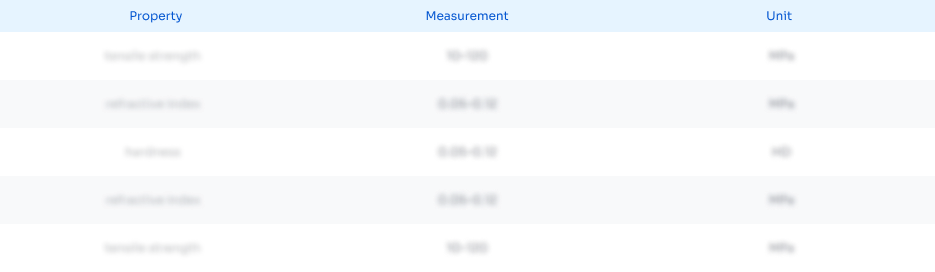
Abstract
Description
Claims
Application Information
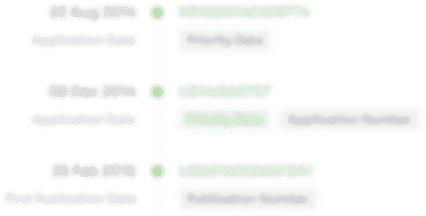
- R&D Engineer
- R&D Manager
- IP Professional
- Industry Leading Data Capabilities
- Powerful AI technology
- Patent DNA Extraction
Browse by: Latest US Patents, China's latest patents, Technical Efficacy Thesaurus, Application Domain, Technology Topic, Popular Technical Reports.
© 2024 PatSnap. All rights reserved.Legal|Privacy policy|Modern Slavery Act Transparency Statement|Sitemap|About US| Contact US: help@patsnap.com