Electromechanical device neural network failure trend prediction method
A neural network and trend forecasting technology, applied in biological neural network models, testing of machine/structural components, measuring devices, etc. The effect of improving reliability and simplifying the amount of calculation
- Summary
- Abstract
- Description
- Claims
- Application Information
AI Technical Summary
Problems solved by technology
Method used
Image
Examples
Embodiment Construction
[0011] The present invention will be described in detail below in conjunction with the accompanying drawings and embodiments.
[0012] Such as figure 1 As shown, the present invention uses a dynamic neural network model for prediction, uses information entropy weighting to perform information fusion on input vibration signals representing equipment operation, and then obtains a consistent description of the operation status of electromechanical equipment. While establishing weighting based on information entropy, considering the impact of time factors on network input, a dynamic neural network prediction model with innovation weighting is established. In the prediction of dynamic neural network, the golden section method is used to determine the optimal number of nodes in the hidden layer. Realize efficient prediction of equipment operation status. The specific steps are as follows:
[0013] (1) Obtain a section of continuous vibration signal data output by a fault-sensitive...
PUM
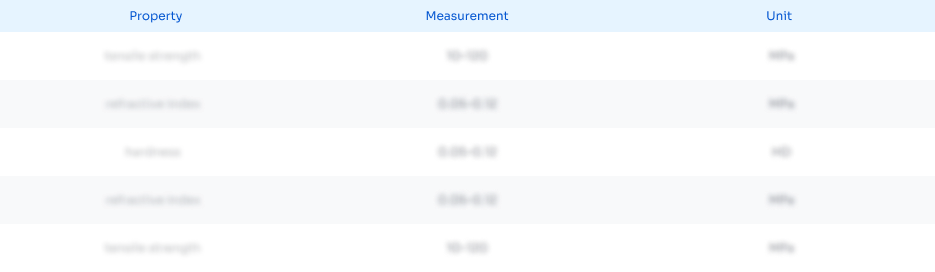
Abstract
Description
Claims
Application Information
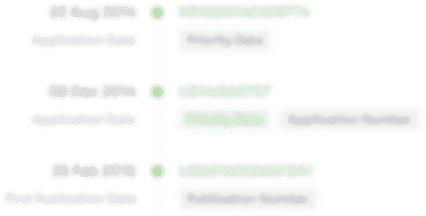
- R&D Engineer
- R&D Manager
- IP Professional
- Industry Leading Data Capabilities
- Powerful AI technology
- Patent DNA Extraction
Browse by: Latest US Patents, China's latest patents, Technical Efficacy Thesaurus, Application Domain, Technology Topic, Popular Technical Reports.
© 2024 PatSnap. All rights reserved.Legal|Privacy policy|Modern Slavery Act Transparency Statement|Sitemap|About US| Contact US: help@patsnap.com