Compressive sensing reconstruction method based on pseudo-inverse adaptive matching pursuit
An adaptive algorithm and compressed sensing technology, applied in image data processing, 2D image generation, calculation, etc., can solve unreachable problems
- Summary
- Abstract
- Description
- Claims
- Application Information
AI Technical Summary
Problems solved by technology
Method used
Image
Examples
Embodiment Construction
[0038] Now in conjunction with embodiment, accompanying drawing, the present invention will be further described:
[0039] The hardware environment used for implementation is: Pentium-43G computer, 2GB memory, 128M graphics card, and the running software environment is: Mat1ab7.0 and windowsXP. We have realized the new algorithm proposed by the present invention with Matlab programming language. The image data adopts 512×512 images of Lena, Pepper and Barbara.
[0040] The present invention is specifically implemented as follows:
[0041] Step 1 Projection measurement: For an original image A with a dimension of N×K, select a Gaussian random matrix Φ with a dimension of M×N (M≤N) to perform projection measurement on the image A to obtain a measurement signal matrix Y. Each element of the selected matrix Φ here has a mean of 0 and a variance of Gaussian distribution. The specific process of projection measurement is as follows:
[0042] Y=Φ·A
[0043] Since the dimensio...
PUM
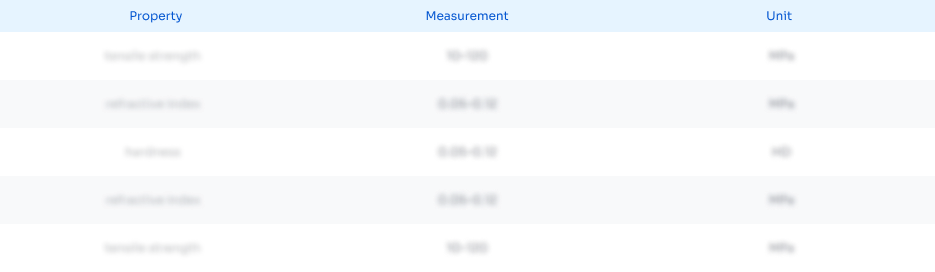
Abstract
Description
Claims
Application Information
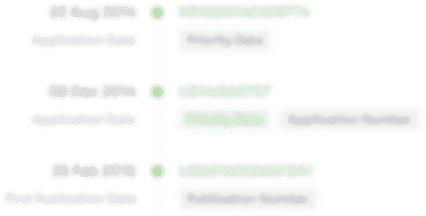
- R&D Engineer
- R&D Manager
- IP Professional
- Industry Leading Data Capabilities
- Powerful AI technology
- Patent DNA Extraction
Browse by: Latest US Patents, China's latest patents, Technical Efficacy Thesaurus, Application Domain, Technology Topic, Popular Technical Reports.
© 2024 PatSnap. All rights reserved.Legal|Privacy policy|Modern Slavery Act Transparency Statement|Sitemap|About US| Contact US: help@patsnap.com